ColibriTD announces H-DES for solving Differential Equations on IBM Quantum Computers
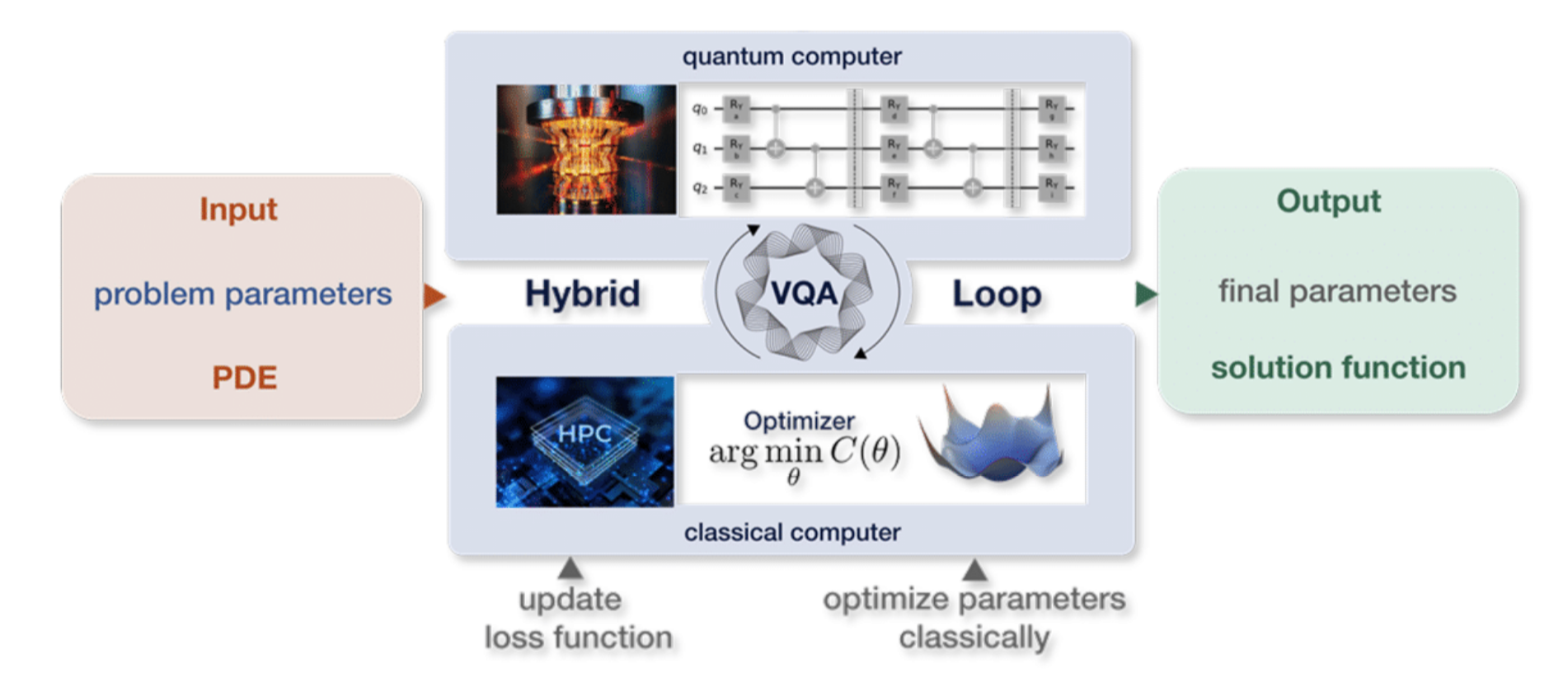
PARIS [25/03/2025] - ColibriTD, a quantum software company developing a platform QUICK (QUantum Innovative Computing Kit), has successfully run their efficient Hybrid Differential Equation Solver (H-DES) on IBM’s cloud-accessible quantum computer powered by the latest IBM Quantum Heron processor. This represents the first successful attempt to solve a partial differential equation (PDE) on a real quantum computer with a Variational Quantum Algorithm.
To make quantum computing broadly accessible, ColibriTD's goal is to develop a user-friendly platform that leverages the power of quantum computing in the noisy intermediate-scale quantum (NISQ) era.The platform QUICK targets users who are accustomed to classical simulation software and want to easily benefit from quantum computers to efficiently simulate multiphysics problems relevant to real-world challenges.
ColibriTD’s universal quantum solver, H-DES, has been developed to solve partial differential equations for modelling industry-relevant use cases, primarily related to fluid dynamics, combustion, mechanics and climate modelling. H-DES’s underlying algorithm is a hybrid (quantum-classical) differential equation solver based on a variational quantum algorithm (VQA) and is applicable to both current and future quantum devices.
Using IBM’s latest, more performant IBM Quantum Heron processor, ColibriTD demonstrated that the H-DES algorithm is robust and could also scale with therapid advancement of IBM’s latest quantum computers.
In the published results, ColibriTD showed how computational fluid dynamics equations were successfully solved with H-DES, demonstrating it can be run successfully on IBM’s quantum systems powered by the latest IBM Heron quantum processor.
The next step of this project will involve the solving of a broader range of more complex PDEs.
These results are a major milestone for ColibriTD and will pave the way towards the large-scale use of quantum technology for multiphysics simulations.
Dr. Laurent Guiraud, Co-Founder and CEO of ColibriTD, commented: "Our latest findings using IBM's Heron QPU mark a significant step towards harnessing the power of quantum computing for solving partial differential equations. This opens up exciting new avenues for research and development infields such as fluid dynamics, materials science and weather forecasting."
Dr. Frédéric du Bois-Reymond, Partner at Earlybird-X, said: “We are super happy about the great work of the team at ColibriTD to enable the relevant use of quantum computing performance solving partial differential equations. This opens strong opportunities to enter big markets with a solid value proposition.”
Access the detailed whitepaper here.
About ColibriTD
ColibriTD is a quantum computing company focused on delivering end-to-end quantum solutions that seamlessly integrate with classical computing infrastructure. Its mission is to make quantum computing accessible to industries seeking cutting-edge solutions for real-world challenges.
For media inquiries, please visit https://www.colibritd.com/
Contact: Dr Laurent Guiraud - laurent.guiraud@colibritd.com
FAQ
(Classical vs Quantum comparison) What is the main result of the whitepaper demonstrating and how does it relate to quantum advantage?
We don’t talk about Quantum Advantage because we don’t claim to have any yet. The goal of this communication was to highlight that we have reached an important step in the direction of quantum utility/advantage. Concerning the comparison to classical method we are preparing to compare to typical use cases provided by multi physics simulation software like OpenFoam and analyze, which equations/problems will benefit the most from our quantum solutions.
(Advantage) What is the main advantage of your algorithm and how do these results provide support in ColibriTD’s long term strategy?
With these results, we proved that today’s quantum computers are stable enough to be used for our generic PDE solver and moreover that our algorithm can solve non-linear partial differential equations. According to our scaling analysis (see white paper section 4) we are close (1-2 years) to tackle PDEs which are interesting for industries, since our algorithm has good scaling properties, such that we can easily apply it to higher dimensional/more complex equations. Thus, combining our scaling analysis with the results on IBM, we can conclude that we approach quantum utility (within a reasonable time frame).
(Limitations) You tested this on 50 qubits in Heron R2. What was stopping you from going further? The state preparation?
Indeed the more qubits we use, the more we have errors on the state preparation and readout errors, as well as other errors. For this equation it was sufficient to use 50 qubits, but we also demonstrated for a more complicated equation 70 qubits + 70qubits. Our algorithm is in principle a low qubit and low depth approach with good scaling properties (section 4 in the white paper). The qubits which are not utilized in the ansatz directly, contribute as ancilla qubits to aid in managing measurement errors. Thus, we have used more qubits in the total solver approach
(Validation score) How do we put an error on the result of the differential equations?How do we perform error propagation from estimated errors on real hardware?
The validation score we defined in the article is used to measure the error on the trial solution of the DEs. For the error propagation, we can estimate the propagation of the gate error by looking at the ansatz.
(Error mitigation) You don’t seem to use QEM in the implementation of your solution. Many recent IBM Eagle/Heron case studies were based on using QEM, like the ones done by Algorithmiq. What’s your views on QEM? It is not applicable or useless in your case? Are there also some scaling limitations with variational algorithms like the one you are using?
We did use little bit of error mitigation/suppression provided by IBM:
https://docs.quantum.ibm.com/guides/configure-error-mitigation
For running on Heron devices, we relied on mitigating errors associated to readout errors treated by twirled Readout Error eXtinction (TREX) measurement twirling and it was sufficient. For Eagle devices we would have needed to use dynamical decoupling or more, but for 50 qubits this would be too time consuming and too costly. Noise, as thermal noise and depolarizing noise can be compensated by the classical optimizer to find the best angles adapted to the noisy environment. We carried out studies on that and could conclude, our algorithm without error mitigation is robust when only dealing with noise at the gate level. The most challenging noise for us is state preparation and readout error, for which we need to still apply error mitigation technqiues.
(Classical optimizer scaling) How about the classical optimizer cost with the problem scale?
There are several strategies how deal with optimizers within VQA on real hardware. Indeed, it is not straight forward to figure out, how the performance these optimizers scale. To guarantee efficient and precise results, we use a combination of optimizer, each for a different purpose. One to initialize fast, followed by a main optimizer (typically global and derivative free), finished by a slow local gradient based optimizer to fine tune and precise the results. Each of them can have different scaling properties. Then we can use symmetries of the problem to manage the number of parameters to optimize. Generally, many of the optimizers we rely on, are used for ML task and they are able handle a lot of parameters and we expect them to scale well for our HDES purposes.
