TQI Exclusive: Moody’s, QuEra Explore Using Quantum Computers to Better Predict Path, Intensity of Tropical Cyclones
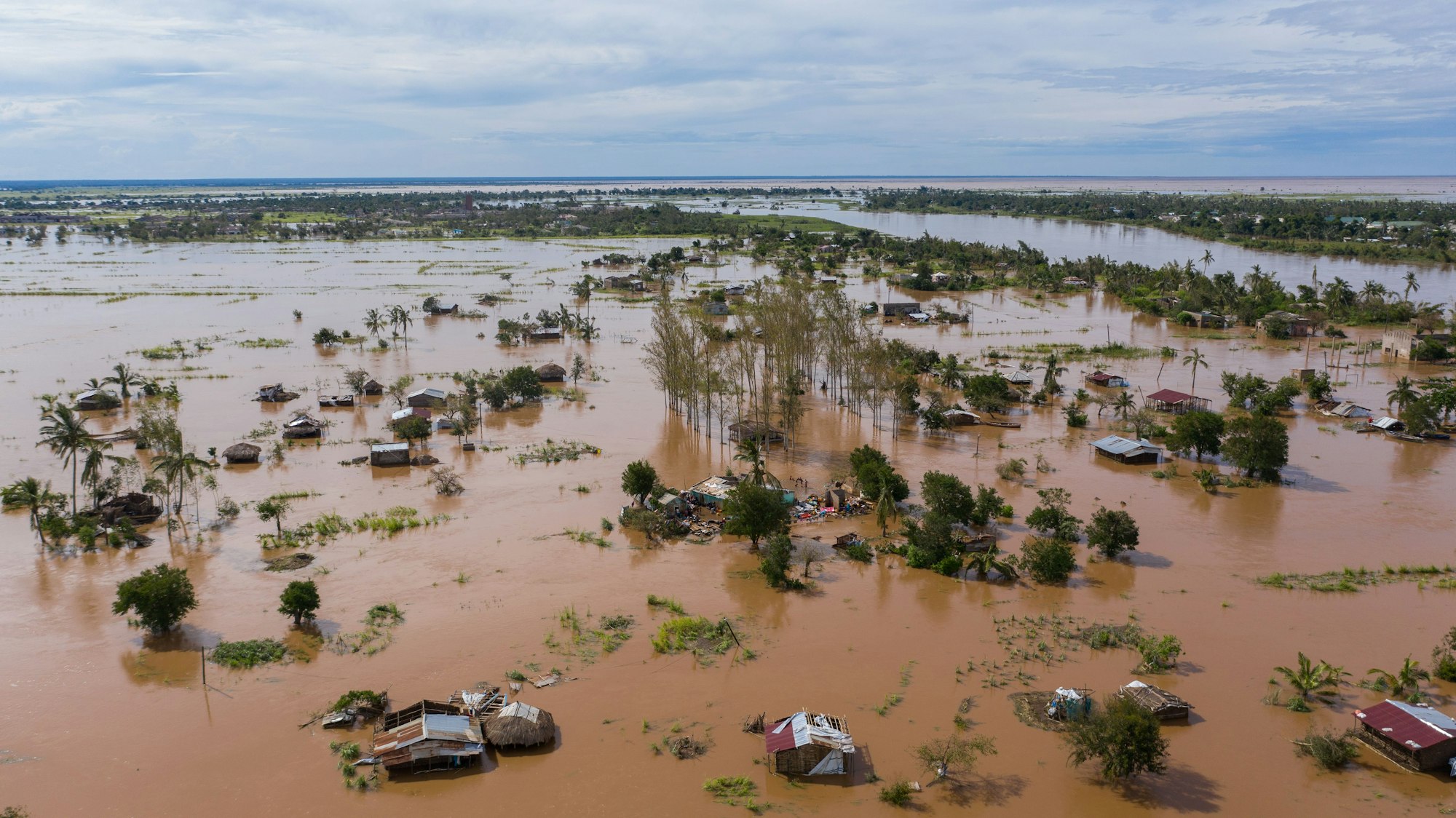
Insider Brief
- A team of researchers from Moody’s is partnering with QuEra to find out whether quantum algorithms can help them explore problem areas that are challenging for purely classical approaches, such as storm forecasting.
- Better prediction of tropical storm strength and direction could help financial analysts predict economic impact, as well as help rescuers and aid workers be better prepared for a disaster.
- The team is exploring ways to build a quantum reservoir computing-based model, capable of processing climate model outputs and storm environment parameters to provide more accurate forecasting.
As use cases begin to take shape for quantum computing, scientists are not just exploring how to use quantum computers to solve difficult challenges, but also how research teams can combine the best of classical with the best of quantum to tackle important problems.
A team of researchers from Moody’s is partnering with QuEra to find out whether quantum algorithms can help them explore problem areas that are challenging for purely classical approaches.
Interestingly, the team is exploring a computational challenge that, on the surface, may seem to have little to do with the financial industry, but is actually of great economic and societal importance. Better prediction of tropical storm strength and direction could help financial analysts predict economic impact, as well as help rescuers and aid workers be better prepared for a disaster.
“There is commercial interest in trying to assess the risk of extreme events, like hurricanes, or high intensity winds, and the potential economic losses, along with human losses, that can derive from this kind of extreme phenomenon,” said Ricardo Garcia, quantum computer engineer, Moody’s Analytics.
In fact, billions of dollars are at stake with each storm. The National Oceanic and Atmospheric Administration (NOAA), to give some context, estimated that Hurricane Katrina – a single storm – caused over $125 billion in damage.
Better forecasts and simulation of tropical cyclone (TC) intensities and their track can significantly improve the quality of Moody’s RMS tropical cyclone modeling suite. According to a Moody’s Analytics blog post, RMS, one of the world’s leading providers of climate and natural disaster risk modeling serving the global property and casualty insurance and reinsurance industries, has helped clients manage their risk during TC events in the North Atlantic for almost 20 years. Real-time TC guidance can significantly impact a company’s financial, operational, and overall solvency state. Moody’s RMS Hwind product helps (re)insurers, brokers, and capital markets understand the range of potential losses across multiple forecast scenarios, capturing the uncertainty of how track and intensity will evolve, the post states.
With the advances in Numerical Weather Prediction (NWP) and new meteorological observations, forecasts of TC movement have progressively improved in global and regional models. However, the accuracy of models in forecasting the intensities of TCs remains challenging for operational weather forecasting and the consequential assessment of weather impacts such as high winds, storm surges, and heavy rainfall.
“From a risk management perspective, the two important parameters are the trajectory and the intensity of the tropical cyclone,” said Carmen Recio Valcarce, Quantum Computing Engineer at Moody’s Analytic. “What we are trying to see is whether we can bias-correct the forecast data that we get from the meteorological agencies.”
As background, conventional TC intensity forecasting, then, mainly relies on three approaches: statistical, dynamical, and statistical-dynamical methods. Better predicting a storm’s intensity at landfall, for example, could give scientists some idea of its humanitarian and economic impact.
Dynamical models, also known as numerical models, are the most complex and typically must rely on high-performance computing (HPC) to solve the physical equations of motion that are part of the highly chaotic atmosphere. While statistical models do not explicitly consider the physics of the atmosphere, they are based on historical relationships between storm behavior and important storm-specific details, such as location and intensity.
The rise of Machine Learning (ML) and Deep Learning (DL) has led to attempts to create better climate modeling and weather forecasting. Recent advances in computational capabilities and the availability of extensive reanalysis of observational or numerical datasets have reignited interest in developing various ML methods for predicting and understanding the dynamics of complex systems.
Reservoir Computing
Scientists realize that, although machine learning and deep learning may lead to better forecasts, ultimately a new approach will be needed to make even more accurate predictions. Neural networks, for example, have been extremely effective for sequence modeling but face challenges. However, because reservoir computing is both more efficient and more stable, the Moody’s scientists believe it may make a better alternative.
The team is exploring ways to build a quantum reservoir computing-based model, capable of processing climate model outputs and storm environment parameters to provide more accurate forecasting, thereby improving short-term and real-time TC risk analysis. Reservoir Computing (RC) is a novel machine-learning paradigm — and it’s particularly suited to quantum computers. Quantum reservoir computing has shown promising results in early non-linear or chaotic time series prediction tests.
“Comprehensive models of climate systems are very costly to run, traditional ML/DL is a strong candidate to reduce the computational workload while achieving similar predictive skill. Nonetheless, they still face several challenges. Physics-informed ML or hybrid techniques are the leading proposal” Garcia said. “On the other hand, reservoir computing posseses a set of unique characteristic that are appealing for building climate surrogate models. Training a RC is fast and stable, it essential amounts to training a linear regression.”
In this approach, a reservoir – or black box – processes the input signals, such as climate time series data, to derive outputs that capture complex patterns, said Garcia. By training a linear regression model on these outputs, the system learns to predict or analyze the data. In the quantum version, the reservoir is replaced with a quantum processor, potentially enhancing the system’s ability to capture intricate details due to the quantum processor’s unique capabilities.
“And now we have access to this high-dimensional system,” said Garcia. “So, you can ask: ‘What can I do with this noisy Hilbert space in terms of helping enhance the learning performance.’ So, there are different directions we can look for to find this ‘advantage.’”
Moody’s QuEra Collaboration
Of course, if you’re going to create a quantum reservoir computing system, you better have access to a good quantum computer. Moody’s researchers decided on a neutral atom quantum device. The neutral atom quantum processing unit – QPU – and the types of quantum simulations it can perform give rise to different quantum reservoirs, the scientists point out. This unique capability can potentially enhance the modeling of tropical cyclone intensity forecasts and data.
The team is collaborating with QuEra Computing, a leading provider of quantum computers based on neutral atoms. In this investigation specifically, the researchers report that the flexible atom arrangements and tunability of optical controls within QuEra’s neutral atom QPU enable the realization of a rich class of Hamiltonians acting as the reservoir.
There are other advantages of the neutral atom approach, for example high qubit density, long coherence times and customizable interactions, making them ideal for complex simulations like tropical cyclone modeling. Their ability to dynamically reconfigure atom positions and perform high-fidelity operations also allows for precise and scalable quantum computations.
Just The Beginning
The research team is clear that this effort is not just a rubber stamp for quantum, or an attempt to try out a novel technology. They are serious about finding real technological solutions for real world problems, said Valcarce.
“We’re not a company that is in the business of quantum computing, so this is good because it gives us an impartial position,” said Valcarce. “We plan to do the benchmarks and the jobs – and when we publish the results, we will publish those results exactly as we see them.”
Despite the challenges of fully exploiting the potential of quantum computers, Valcarce added that the team is cautiously optimistic about quantum.
“The actual problem sizes that we work with end up with estimation of resources that is way beyond what we have today, even in some roadmaps of the quantum providers,” said Valcarce. “So, we have a feeling that we are still quite a few years away, but there’s still excitement because of these kinds of problems where we are currently limited by the very nature of the problems – problems where we have very, very high dimensions, and therefore we are only able to apply approximations to make models tractable.. So quantum opens some doors to further exploring things.”