Technique Injects New Possibilities For Quantum Advantage Using Photonic Quantum Devices
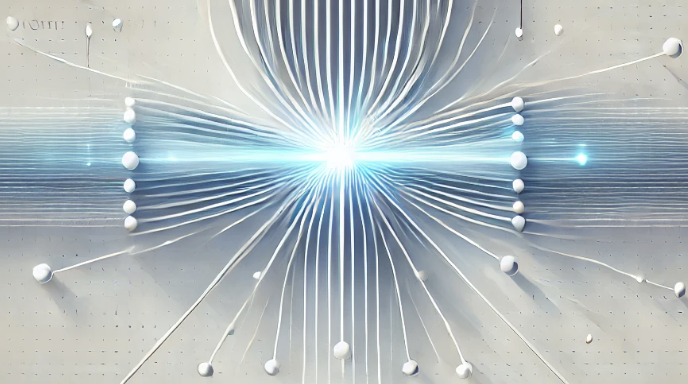
- A recently published study on arXiv explores the use of “state injection” as a method to enhance the control and flexibility of photonic circuits for more advanced quantum computations.
- The researchers suggest that this method could surpass classical algorithms in certain machine learning tasks, showing promise for near-term quantum computing applications.
- As quantum technologies advance, the approach reduces experimental complexity and requires fewer resources compared to adaptive techniques.
Researchers have proposed a new scheme for enhancing photonic quantum devices, leveraging a concept called State Injection (SI), according to a study.
The team’s work, published on the pre-print server arXiv, suggests that SI allows quantum systems to surpass the limits of traditional linear optics models, offering a pathway to increased expressivity in quantum computations, particularly for tasks that are computationally hard for classical systems.
It may also serve as a step toward quantum advantage for certain tasks, the researchers add in the paper.
Expanding Quantum Power with State Injection
According to the team, the development of SI, a quantum computing technique that combines linear optical circuits, is the central contribution of the work. Linear optical circuits are systems that manipulate photons using basic components such as beam splitters and phase shifters, relying on linear transformations without changing the photon states. These circuits are essential in quantum computing for processing quantum information, but their capabilities are limited without non-linear interactions, which restricts the complexity of the tasks they can perform.
SI allows for new quantum states to be injected into a circuit based on measurement outcomes, making quantum models more controllable and expressive. This new method is particularly important because it doesn’t require real-time reconfiguration of the circuit, which is a significant limitation in existing approaches, such as Adaptive Linear Optics (ALO).
The researchers further suggest that SI can increase the capability of quantum devices, allowing them to tackle computational problems that are currently unsolvable by classical computers, especially in machine learning tasks that rely on probability estimation. The work provides both theoretical and practical advances, showing how this method could bridge the gap between near-term quantum devices and fully fault-tolerant quantum computers.
Quantum Photonic Devices and Their Limitations
Quantum computing has long promised significant advantages over classical computing, but, as yet, scientists are still working on achieving universal, fault-tolerant quantum systems. One of the most promising platforms for quantum computing is photonic systems, which use light particles (photons) to perform quantum computations. However, the current state of photonic quantum devices faces several challenges, including the need for adaptive measurement-based operations and access to large numbers of modes and coherent photons.
Sub-universal quantum models, such as Boson Sampling and Gaussian Boson Sampling, have shown some promise in providing intermediate computational advantages. However, their range of solvable problems is limited, and the scalability of these systems remains a hurdle.
State Injection as a Non-linear Quantum Tool
The researchers propose that SI provides a practical solution for enhancing the expressivity of quantum photonic devices — or their ability to represent and process complex information — without the need for complex real-time adjustments. In a typical linear optical circuit, the input state, usually a Fock state, is processed through a series of unitary operations, and adaptive measurements are made along the way. SI introduces the ability to measure certain modes and then re-inject quantum states into the system based on the outcomes of these measurements, effectively creating a feedback loop that enhances the circuit’s expressivity.
SI differs from the Adaptive Linear Optics (ALO) scheme in that it does not require the circuit to be reprogrammed after each measurement. Instead, the parameters of the unitary operations are preset, which simplifies the experimental setup. This reduction in complexity makes SI more feasible for near-term quantum devices.
One of the key technical achievements in this work is the demonstration that SI can increase the controllability of the quantum states produced by the circuit. The researchers quantify this by showing how the number of degrees of freedom (DoF) of the output state increases when SI is used, compared to a standard linear optical circuit.
Machine Learning and Quantum Algorithms
One of the potential applications for SI is in the field of quantum machine learning. Machine learning tasks often require non-linear transformations of data, and the researchers demonstrate how SI can provide the necessary non-linearity to enhance the performance of quantum algorithms in tasks such as probability estimation. Specifically, they show that their method can open up a pathway to potential quantum advantage over classical algorithms in certain machine learning tasks. If the method scales and is confirmed, that would make it a valuable tool for near-term quantum computing applications.
In addition to quantum machine learning, SI could be useful in a wide range of quantum computing applications, including optimization problems and quantum simulations. The fact that SI does not rely on real-time reconfiguration makes it particularly well-suited for platforms that have limited computational resources.
Theoretical Insights:
The researchers also provide theoretical results on the evolution of the purity of the quantum states generated by SI circuits. Purity is a measure of how mixed a quantum state is; pure states are more distinguishable, while mixed states lose some of their quantum information. The team shows that while SI increases the controllability of the quantum model, it can also reduce the purity of the output states. However, they argue that this trade-off is manageable and that the overall benefits of increased expressivity outweigh the loss in purity.
The paper also explores the impact of SI on the distinguishability of quantum circuit outputs, which is an important factor in determining the success of quantum algorithms. By carefully selecting the injection functions (the rules that govern how new quantum states are injected into the system), the researchers show that it is possible to maintain a high degree of distinguishability while still benefiting from the increased controllability provided by SI.
Limitations and Future Directions
While the researchers present a compelling case for the advantages of SI, there are still some limitations to their approach. For example, the reduction in purity could be a challenge for certain quantum algorithms that rely on highly pure states. Additionally, the experimental implementation of SI will require further development, particularly in terms of the photon-counting measurements needed to make the scheme work in practice.
Future research will focus on expanding the SI framework to more complex quantum systems, such as many-body systems. The team is also interested in exploring how SI could be integrated with other quantum platforms, such as superconducting qubits, to create hybrid quantum systems with enhanced computational power.
The research was conducted by a team of scientists including Léo Monbroussou and Eliott Z. Mamon from Sorbonne Université, Hugo Thomas from Quandela, Verena Yacoub from Sorbonne Université, Ulysse Chabaud from DIENS, Ecole Normale Supérieure, and Elham Kashefi from University of Edinburgh.