Taking Quantum Computers to Mars: Researchers Use D-Wave Device to Guide Interplanetary Mission Planning
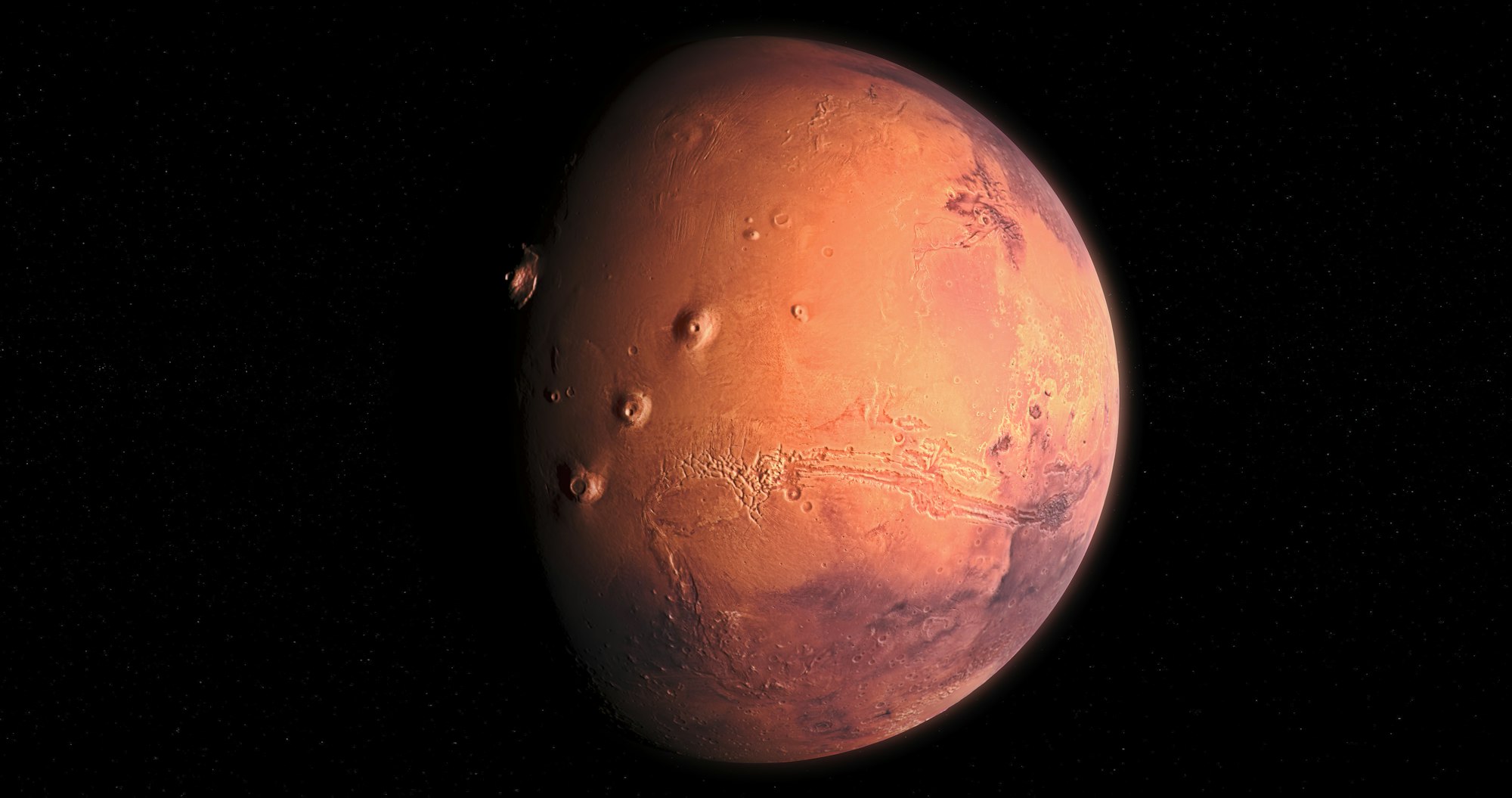
Insider Brief
- A study demonstrated how quantum annealing, combined with classical methods, can optimize spacecraft trajectories, focusing on a low-thrust Earth-to-Mars mission.
- Hybrid solvers proved more accurate and robust than fully quantum solvers, though current quantum hardware limits scalability and solution precision.
- Future improvements in quantum hardware, embedding techniques and optimization methods could expand quantum annealing’s role in space mission planning.
A study in Astrodynamics has revealed how quantum annealing, a technique within quantum computing, can address spacecraft trajectory optimization. The researchers applied their method to a low-thrust Earth-to-Mars transfer, showcasing the potential of hybrid quantum-classical solvers to enhance space mission planning.
While the results are promising, there is still work to do — and a need for hardware improvements — before quantum and hybrid classical-quantum approaches displace traditional methods in solving these tricky optimization problems, according to the paper.
Hybrid Solvers
The study, led by scientists from Sapienza University of Rome, reformulated the trajectory optimization problem into a format suitable for quantum annealers. Using D-Wave’s quantum systems, they found that hybrid solvers — those combining quantum and classical computational resources — could produce trajectory solutions comparable to traditional methods. These solvers reliably converged even when starting from suboptimal initial guesses, offering a degree of robustness that could be critical for real-world applications.
Fully quantum solvers, which rely solely on quantum annealing, demonstrated faster processing per iteration than hybrid approaches. However, they required significantly more iterations to approach a solution, struggled with problem complexity and failed to converge to the same level of accuracy, according to the paper. Despite these challenges, the speed of quantum annealers in individual computations suggests potential for scaling as hardware improves.
Optimized trajectories are vital for reducing mission costs, minimizing fuel consumption and enabling more complex exploratory missions. The team suggests that the research validates quantum annealing as a future tool for these tasks but underscores the need for technological advancements in quantum hardware and embedding techniques.
Spacecraft Trajectory
Spacecraft trajectory optimization is a fundamental task in aerospace engineering, involving the calculation of an efficient path while minimizing resources like fuel. Traditional approaches typically involve calculus-based methods or numerical optimization, both of which can be computationally expensive as problem complexity grows. The nature of these problems fit the natural strengths of quantum and hybrid quantum-classical computation.
In this study, the researchers employed a transcription procedure to convert the trajectory problem into a quadratic unconstrained binary optimization (QUBO) format required by quantum annealers. This involved discretizing the trajectory with a pseudospectral method, which approximates the spacecraft’s path as a series of algebraic constraints. Essentially, this means the researchers broke down the spacecraft’s path into manageable points and used math to connect them, making the problem easier for computers to solve.
The test case focused on a simplified scenario of a trip from Earth to Mars — or Earth-to-Mars transfer. Assumptions included circular orbits and a fixed flight time, which reduced the problem size to accommodate the limitations of current quantum annealers. The hybrid solver combined classical preprocessing to simplify the problem before leveraging quantum annealing, while the fully quantum solver directly relied on the annealing process.
Fewer Iterations
According to the team, the hybrid solver outperformed the fully quantum solver in both accuracy and robustness. It required fewer iterations — typically six compared to the 55 iterations needed by the fully quantum approach. Optimization times for the hybrid solver averaged around three seconds per iteration, with minimal variation, while fully quantum iterations were faster at 54 milliseconds each. However, the quantum solver’s need for far more iterations negated much of its speed advantage.
Hybrid solutions also achieved lower final errors in critical parameters like position and velocity, ensuring the spacecraft’s trajectory closely met the mission objectives. By comparison, the quantum-only solver produced less precise results, highlighting the limitations of current quantum hardware in handling high-dimensional and complex problems.
Cost metrics, such as fuel consumption, further emphasized the superiority of hybrid solvers. The hybrid approach consistently minimized fuel usage, a critical factor for optimizing interplanetary missions.
Limitations
The scalability of current quantum annealers remains a significant barrier, the paper suggests. The D-Wave Advantage system, used in the study, can handle approximately 5,000 qubits, but embedding complex problems onto the annealer’s architecture often requires far more resources. Embedding techniques, which create additional connections between qubits to represent the problem, increase susceptibility to noise and errors, particularly when the chains of linked qubits become long.
Additionally, the discrete nature of the QUBO representation limits solution accuracy. Problems requiring higher precision or involving mixed variables (both binary and continuous) are particularly challenging for quantum annealers.
Future Directions — To The Moon?
Despite the limitations, the research marks a critical step forward in applying quantum computing to aerospace engineering challenges. Hybrid solvers are already demonstrating practical potential, offering robust and efficient solutions that rival traditional methods in some scenarios. However, the above mentioned limitations of fully quantum solvers highlight the need for more work — which, the study suggests, prompts several interesting avenues for future research that scientists could explore in what might be a more and more important task — guiding spacecraft missions.
First, quantum hardware must improve to handle larger, more complex problems, with advances needed in qubit connectivity and coherence. Developing more efficient embedding algorithms could also allow more intricate problems to be represented without overwhelming available resources.
Researchers could also explore alternative optimization methods that integrate continuous and binary variables more seamlessly. Extending the framework to more complex scenarios, such as multi-planet trajectories or variable thrust profiles, could further demonstrate the utility of quantum annealing.
The transcription procedure itself could be refined to achieve greater sparsity in the QUBO formulation, improving computational efficiency. Additionally, applying other quantum algorithms, such as constrained quadratic models, might reduce the need for certain simplifications.
Ultimately, as future work on algorithm develops and as quantum computing hardware matures, its application to space exploration could expand, enabling faster and more cost-effective mission planning.
Here’s a complete list of the team: The study was led by Federico De Grossi, Andrea Carbone and Christian Circi from Sapienza University of Rome. Dario Spiller represented the School of Aerospace Engineering in Rome. Additionally, Daniele Ottaviani and Riccardo Mengoni contributed from CINECA, a leading supercomputing center located in Casalecchio di Reno, Bologna, Italy.
The paper itself is technical and in-depth and can provide more details that this story can provide. Please read the paper here for a deeper dive.