Researchers Say Quantum Machine Learning, Quantum Optimization Could Enhance The Design And Efficiency of Clinical Trials
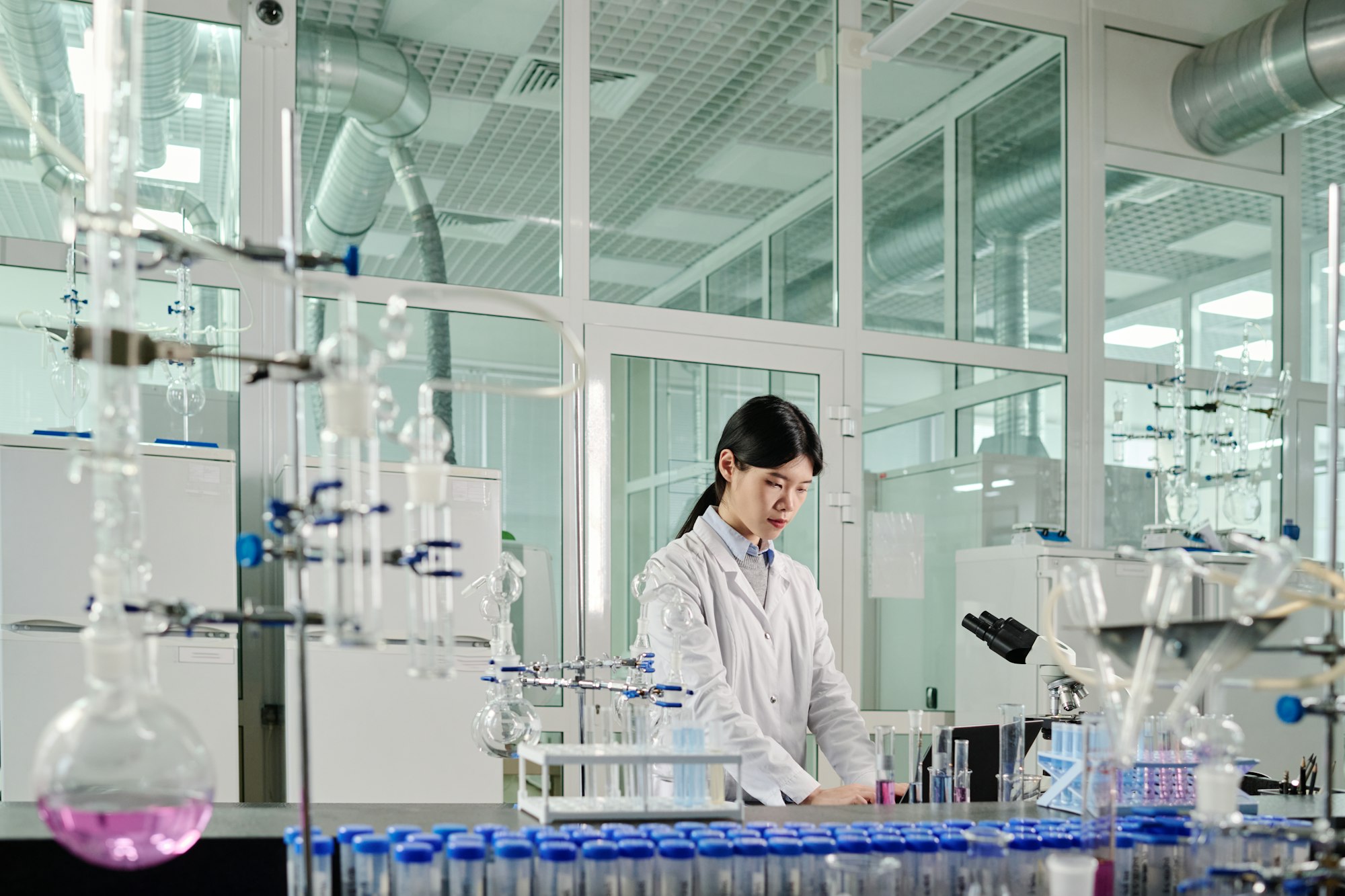
Insider Brief
- A new study explores how quantum optimization and quantum machine learning (QML) can be applied to improve clinical trial design, site selection and cohort identification.
- Quantum algorithms offer the potential to enhance trial simulations, particularly in predicting drug effects and optimizing site selection, by efficiently handling complex, high-dimensional data that classical systems struggle with.
- Despite the promise of these technologies, the study acknowledges that current quantum hardware limitations, such as noise and lower gate fidelity, pose challenges, making hybrid quantum-classical approaches the most viable option for now.
Better, more efficient drug design is often touted as a key use case for quantum computers.
Now, a recent study suggest that beyond the drug-development stage, robust quantum computers could be used to improve the design and execution of clinical trials, addressing some of the key challenges that have long plagued the process, including delayed timelines, recruitment issues and data reliability. Further, while quantum computing is still in its early stages, the study suggests that even with current limitations, quantum algorithms can offer significant improvements in clinical trial design.
Published in Trends in Pharmacological Studies, the study specifically points to how quantum optimization algorithms and quantum machine learning (QML) — and hybrid quantum-classical variations — could affect the clinical trial landscape. By improving the accuracy of trial simulations and optimizing key components like site selection and cohort identification, quantum computing promises to accelerate trial timelines and enhance their overall efficiency.
Quantum Computing and Trial Optimization
Clinical trials are notorious for their lengthy timelines, often spanning years, and they face numerous obstacles along the way, according to the research team, which included scientists from IBM and GNQ Insilico.
One of the most promising applications of quantum computing discussed in this study is its ability to optimize trial design, particularly through quantum optimization algorithms like the Quantum Approximate Optimization Algorithm (QAOA). Quantum optimization leverages the power of quantum computers to solve complex problems faster by exploring multiple possibilities simultaneously, while quantum machine learning (QML) enhances machine learning algorithms by using quantum systems to process large datasets more efficiently.
These algorithms offer an advanced method for clinical trial-related activities, such as selecting trial sites, simulating patient outcomes and refining cohort selection—all areas where traditional approaches have shown limitations. Quantum computing’s ability to process complex datasets faster and more efficiently than classical computing offers a potential solution to these challenges. It could also open up new possibilities for more equitable trials compared to ones designed by classical — even classical machine learning (ML) — computers.
The team writes, for example: “Despite the progress made with ML in clinical trials, issues such as the lack of explainability of ML decisions remain a concern. The ‘black-box’ nature of many ML models can hinder their acceptance by regulatory bodies and medical professionals who require transparent decision-making processes to validate and trust the outcomes of clinical trial.”
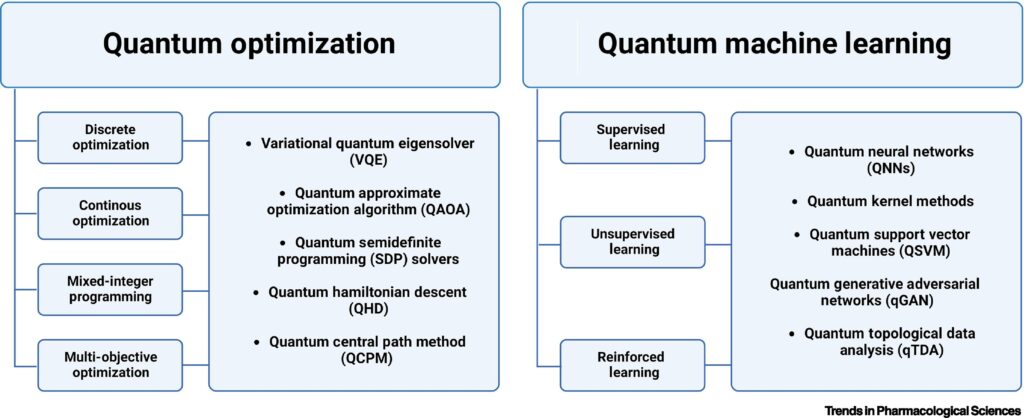
Simulations and Site Selection
According to the researchers, quantum computers also have the potential to outperform classical methods in simulating the pharmacokinetics and pharmacodynamics (PBPK/PD) of drugs. These simulations, which predict how a drug will behave in different patient populations, are critical for determining the safety and efficacy of treatments. For example, they can help researchers understand how a drug interacts with the body, including drug absorption, distribution, metabolism and excretion.
Quantum algorithms can theoretically process these models more accurately, accounting for the intricate biological and physiological factors that are often missed in classical models. Currently, classical models often fail to capture the full complexity of these interactions, leading to discrepancies between preclinical and clinical trial results, the researchers write, adding that quantum computing, with its ability to handle large-scale computations, offers a more precise way to model these interactions and predict drug outcomes in real-world populations.
Quantum optimization algorithms also have the potential to transform how trial sites are selected. Site selection is one of the most crucial aspects of clinical trials, as the success of a trial depends heavily on finding the right location with access to the appropriate patient population. Traditionally, this process has been carried out manually or through rule-based systems. These systems use predefined rules and logic to make decisions or solve problems, relying on expert knowledge rather than learning from data like modern machine learning models. According to the researchers, rule-based systems, while helpful, are not always efficient. Quantum computing can improve this by using advanced optimization algorithms to analyze vast datasets, identifying the best sites based on a combination of infrastructure, patient demographics, and regulatory factors.
Additionally, cohort identification — determining which patients are eligible for a trial — is another area where quantum algorithms can make a difference. In clinical trials, defining the right patient cohort is key to ensuring the success of the trial. Classical methods often rely on rule-based systems, which can be time-consuming and imprecise. Quantum algorithms, particularly QML, could one day process complex patient data to identify optimal cohorts more quickly and accurately, leading to better trial outcomes.
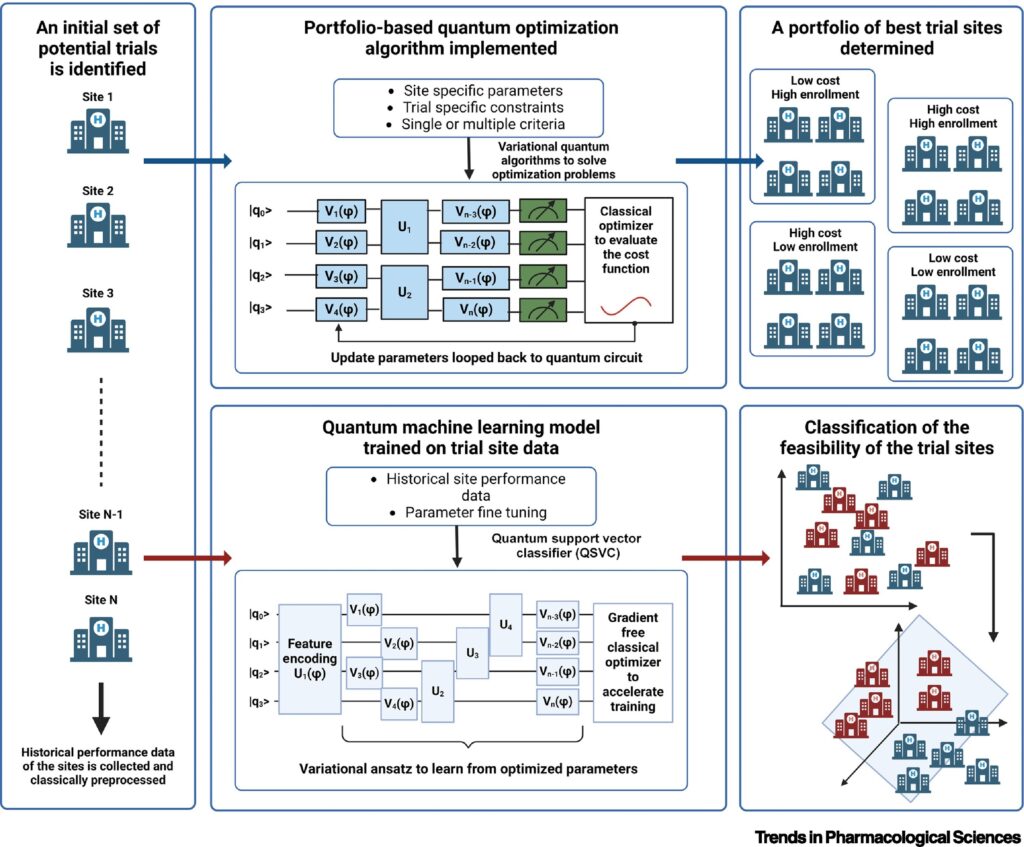
Broader Quantum Capabilities in Healthcare
While the primary focus of the study is on quantum optimization and QML, it also touches on the broader capabilities of quantum computing in healthcare. Quantum computing excels in solving both linear and non-linear systems of equations, which are common in the simulation of drug interactions. These capabilities make it a powerful tool for advancing drug discovery and personalized medicine. Moreover, quantum computing has the potential to enhance machine learning models used in clinical trials, particularly when it comes to processing large, complex datasets such as electronic health records (EHR).
To conduct the study, the team embarked on a detailed examination of current quantum computing techniques, including the use of quantum error correction to reduce noise and improve the reliability of quantum systems. The authors also explore the potential of hybrid quantum-classical algorithms, which combine the strengths of both quantum and classical computing to solve complex optimization problems more efficiently.
Limitations and Future Directions
Despite the promising findings, the study acknowledges several limitations of quantum computing. One of the primary challenges is hardware noise, which can reduce the accuracy of quantum computations. Although error correction methods are improving, quantum computing has not yet reached the level of fault tolerance needed for widespread commercial use. Additionally, the study notes that while quantum computing has shown promise in PBPK/PD modeling and site selection, further research is needed to fully realize its potential in these areas.
Looking ahead, the study suggests several future directions for research. One of the key areas for improvement is the integration of quantum algorithms with existing clinical trial infrastructure. This will require collaboration between researchers, pharmaceutical companies and regulators to ensure that quantum computing can be effectively applied in real-world clinical settings. Additionally, the study calls for more work on developing quantum algorithms that can handle the inherent variability in biological data, particularly in genomics and personalized medicine.
The research was conducted by a team from several prominent institutions. Hakan Doga, Aritra Bose, and Laxmi Parida are from IBM Research and IBM Quantum. M. Emre Sahin is affiliated with The Hartree Centre, STFC, while Joao Bettencourt-Silva is based at IBM Research, Dublin, Ireland. Anh Pham, Eunyoung Kim, Anh Pham, Eunyoung Kim and Alan Andress are from Deloitte Consulting LLP. Sudhir Saxena and Radwa Soliman are from GNQ Insilico Inc. Jan Lukas Robertus is affiliated with Imperial College London and Royal Brompton and Harefield Hospitals and Hideaki Kawaguchi is from Keio University. Finally, Daniel Blankenberg is from the Lerner Research Institute, Cleveland Clinic.