Quantum Machine Learning Advances: Researchers Achieve 95.8% Accuracy in Continual Learning with Superconducting Quantum Processor
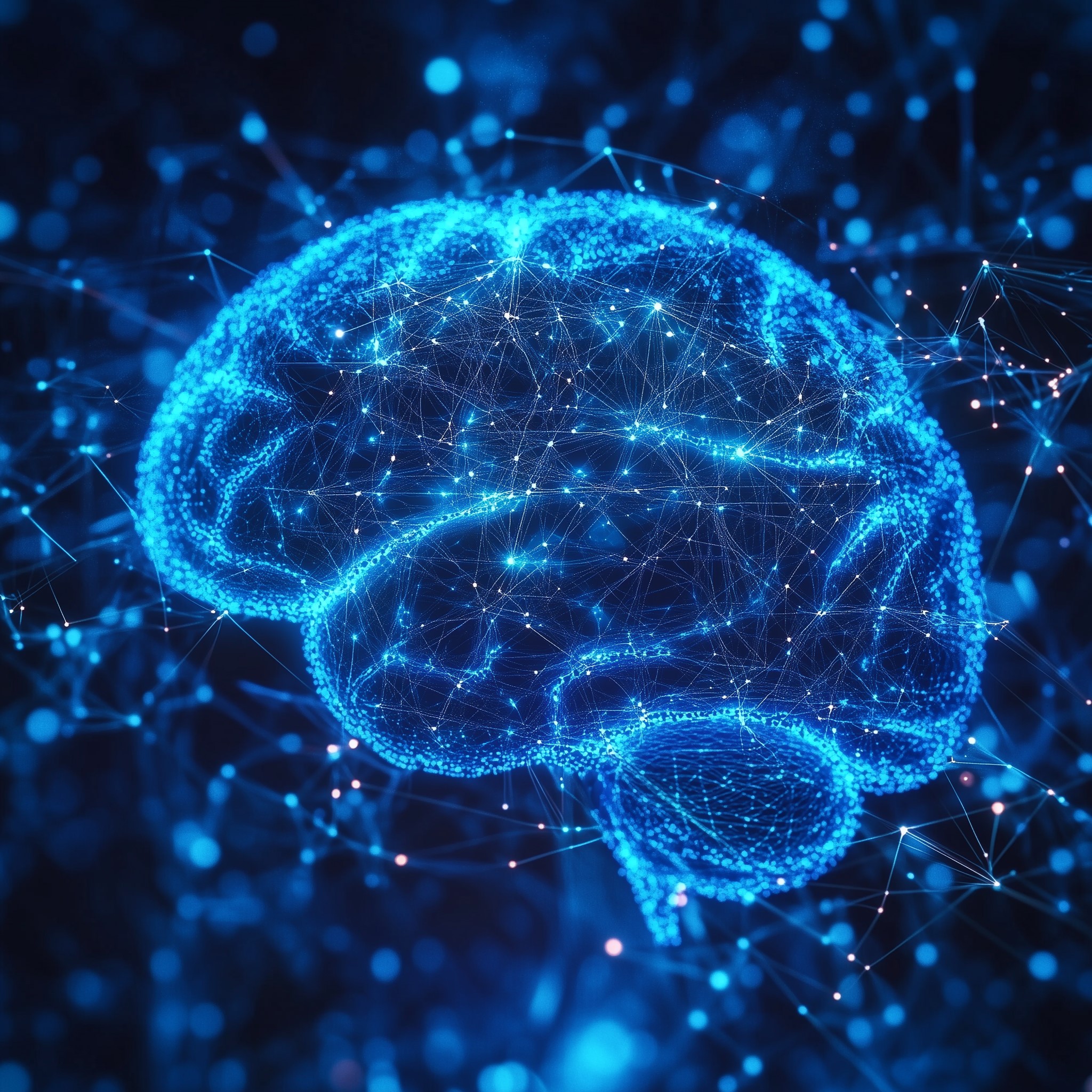
Insider Brief:
- Researchers from Zhejiang University and Tsinghua University demonstrated quantum continual learning on a fully programmable superconducting quantum processor, addressing the challenge of catastrophic forgetting in machine learning.
- The team used an Elastic Weight Consolidation (EWC) strategy to balance memory retention and learning flexibility, successfully training a quantum classifier on three tasks: Fashion-MNIST image classification, MRI scan identification, and quantum state recognition, achieving an average accuracy of 92.3% across these tasks specifically.
- Without EWC, the quantum system experienced performance loss on earlier tasks, but EWC allowed the classifier to retain high accuracy across all tasks.
In a recent study, researchers from Zhejiang University and Tsinghua University have demonstrated quantum continual learning on a fully programmable superconducting quantum processor. This is a notable development in quantum machine learning, where the challenge of catastrophic forgetting—common in classical machine learning—has long hindered progress.
A New Frontier for Quantum AI
Continual learning, also known as incremental or lifelong learning, refers to the ability of a system to learn new tasks sequentially without forgetting previously acquired knowledge. This has been a fundamental challenge for AI, especially with deep learning systems, where learning a new task often leads to the loss of performance on previous tasks. The research team addresses this issue in quantum machine learning, using the power of quantum systems to demonstrate the possibility of continual learning in a quantum framework.
To amend the damage done by catastrophic forgetting, the team implemented an Elastic Weight Consolidation (EWC) strategy. EWC balances memory retention with learning flexibility by penalizing changes to the most important parameters for previously learned tasks, ensuring the system can learn new tasks while preserving knowledge from earlier ones. Using their superconducting quantum processor, the team sequentially trained a quantum classifier on three tasks: classifying real-life images from Fashion-MNIST, identifying medical images from MRI scans, and recognizing quantum states. The quantum classifier achieved an impressive 92.3% average accuracy across these tasks, surpassing the classical counterparts, with fewer computational resources.
Key Advances in Quantum Learning
As noted in the article, one of the central achievements of this study is the implementation of EWC. The researchers demonstrated that, without the use of EWC, the quantum classifier suffered from catastrophic forgetting, as its performance on previously learned tasks sharply declined when new tasks were introduced. However, by applying EWC, the quantum system was able to retain high performance across all tasks, relevant for a dynamic learning environment.
In addition to solving catastrophic forgetting, the quantum system attained an overall accuracy of 95.8% on continual learning tasks, outperforming the classical neural network, which achieved only 81.3%. This performance was achieved despite the quantum model having fewer parameters than the classical one.
The study not only provides an example of the potential advantage of quantum systems in continual learning scenarios but also presents a notable comparison between quantum and classical models. The quantum classifier’s ability to retain knowledge and adapt to new tasks with higher accuracy and efficiency is a positive omen for the future of quantum machine learning.
Toward Quantum Artificial Intelligence
By enabling quantum systems to handle dynamic and non-stationary data streams through continual learning, the article notes that this research opens the door for future applications in fields such as autonomous systems, medical diagnostics, and complex scientific simulations. Though challenges remain—such as extending quantum continual learning to unsupervised and reinforcement learning—this study provides a solid foundation for future advancements in quantum machine learning.
The authors who completed the study include Chuanyu Zhang, Zhide Lu, Liangtian Zhao, Shibo Xu, Weikang Li, Ke Wang, Jiachen Chen, Yaozu Wu, Feitong Jin, Xuhao Zhu, Yu Gao, Ziqi Tan, Zhengyi Cui, Aosai Zhang, Ning Wang, Yiren Zou, Tingting Li, Fanhao Shen, Jiarun Zhong, Zehang Bao, Zitian Zhu, Zixuan Song, Jinfeng Deng, Hang Dong, Pengfei Zhang, Wenjie Jiang, Zheng-Zhi Sun, Pei-Xin Shen, Hekang Li, Qiujiang Guo, Zhen Wang, Jie Hao, H. Wang, Dong-Ling Deng, and Chao Song.