Quantum Lenses: ESA Explores Earth Observation Insights Through Hybrid Quantum AI Models
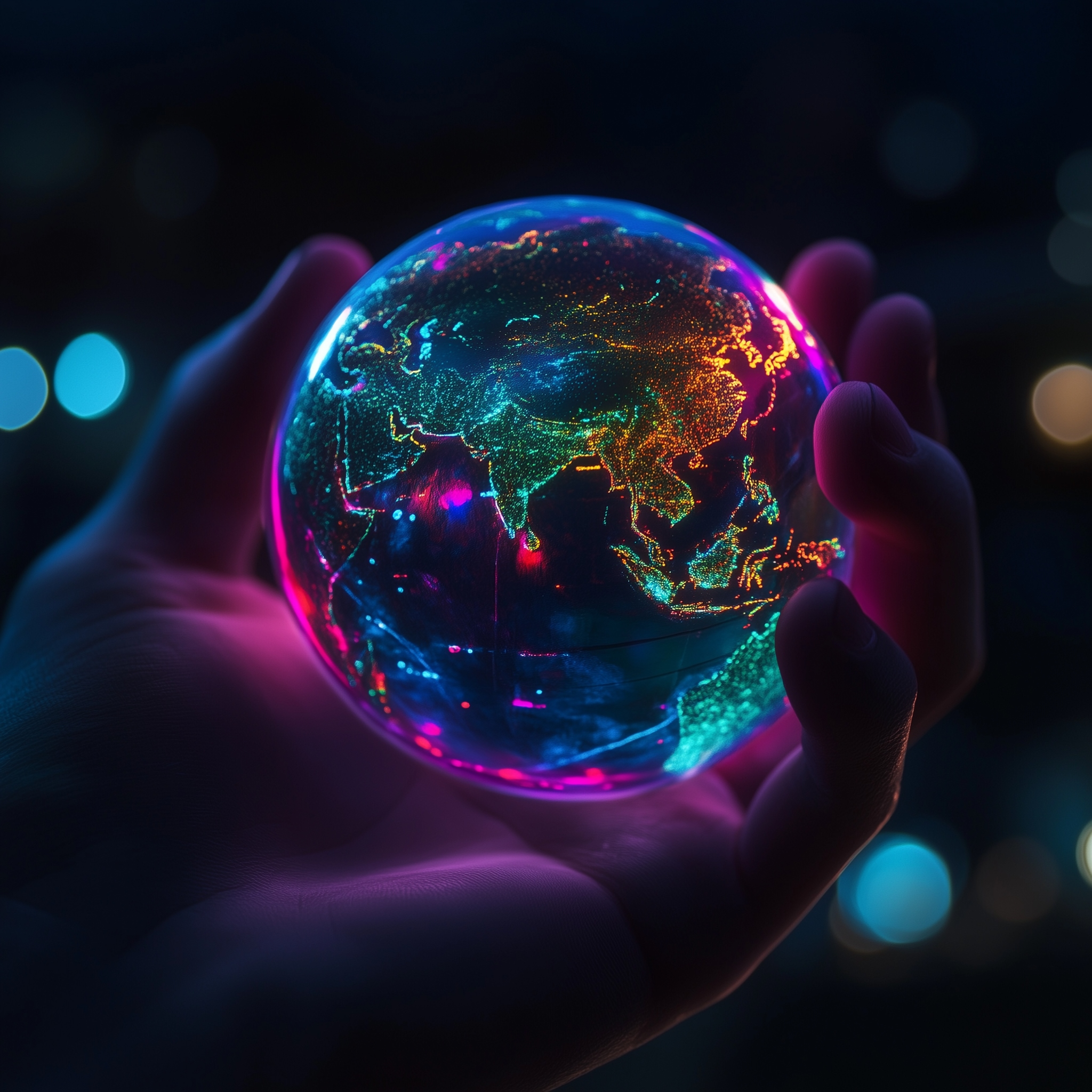
Insider Brief:
- Earth Observation (EO) is important in monitoring environmental changes and natural disasters, but the vast amount of satellite data presents challenges in efficient processing and analysis.
- Researchers from the European Space Agency and Sapienza University of Rome are exploring hybrid quantum neural networks to improve the computational efficiency and accuracy of machine learning tasks in EO.
- The study evaluated two quantum computing frameworks, Qiskit and PennyLane, and found that both delivered strong performance, with PennyLane demonstrating faster convergence due to its integration with machine learning libraries like PyTorch.
- While quantum-enhanced models showed promise, the research highlighted challenges related to quantum hardware limitations and model stability, indicating the need for further refinement to fully realize the benefits of hybrid QNNs in EO applications.
Earth Observation (EO) is an essential tool used in monitoring and understanding our planet, providing insights into environmental changes, land use, and natural disasters. However, the vast amount of data collected through satellites presents a challenge around how to efficiently process and analyze these complex datasets to extract meaningful information. Traditional deep learning models have been widely used, but as the demand for higher accuracy grows, their limitations are apparent. In response, researchers are turning to quantum computing as a potential way to overcome these barriers.
In a new preprint published on arXiv, researchers from the European Space Agency and the Sapienza University of Rome explore how hybrid quantum neural networks, which integrate quantum computing with deep learning, may be used for machine learning tasks within EO applications to improve computational efficiency and accuracy.
Investigating Quantum Libraries and Model Performance
Artificial neural networks have become central to machine learning due to their ability to model complex relationships in large datasets. Earth Observation tasks, where vast amounts of satellite data need to be processed efficiently, integrating quantum computing into neural networks may provide an additional advantage. Traditional methods, while effective in tasks like image recognition, often face challenges when scaling to larger datasets. According to the study, hybrid quantum neural networks could help address these limitations, providing benefits when applied to the large-scale datasets typical in EO.
A key aspect of the research was evaluating the performance of different quantum computing libraries used to train these hybrid models. The research focused on two popular quantum frameworks: Qiskit, developed by IBM, and PennyLane, developed by Xanadu. Both were examined for their computational efficiency and effectiveness in integrating quantum components with traditional neural networks.
The results showed that both Qiskit and PennyLane delivered strong performance, though there were subtle differences in accuracy and convergence speed. PennyLane, due to its seamless integration with widely-used machine learning libraries such as PyTorch, demonstrated faster convergence, allowing for more efficient development of hybrid models. Meanwhile, Qiskit proved reliable, with only minor variations in accuracy across the tested model.
Stability, Sensitivity, and New Architectures in Hybrid Models
Another central focus of the research was an exploration of the stability and sensitivity of hybrid quantum neural networks to different initialization values, particularly how these starting conditions influence model performance. The study emphasized the importance of understanding how initialization impacts the stability and training efficacy of both quantum and classical models.
By comparing the stability and convergence behaviors of classical and quantum-enhanced models under various initialization settings, the researchers found that quantum models generally exhibited higher accuracy and more stable performance. While some quantum models did show increased variance, the integration of quantum circuits consistently led to performance improvements, highlighting the potential of quantum computing in enhancing neural network training.
The study also introduced a new architectural approach by incorporating quantum circuits into vision transformers, a model type that uses self-attention mechanisms for image processing. According to the researchers, these hybrid quantum vision transformers represent the first application of quantum-enhanced ViTs to Earth Observation (EO) tasks.
By combining classical convolutional layers with quantum-enhanced components, the researchers explored whether hybrid models could improve classification tasks. The results showed that HQViTs achieved slightly higher accuracy than their classical counterparts, suggesting that even the integration of simple quantum circuits may positively impact model performance.
Challenges and Future Directions
While the potential of hybrid quantum neural networks in Earth Observation has potential, the study acknowledges several challenges that must be addressed before these models can be practically deployed. One of the primary hurdles is the current limitations of quantum computing hardware, such as high error rates, limited coherence times, and scalability issues. These constraints hinder the full exploitation of quantum capabilities in real-world applications. Additionally, quantum circuits, while showing potential in improving model accuracy, introduce variability in performance, as seen in the increased variance in some models. This variability indicates that more work is needed to stabilize hybrid models and fine-tune the integration of quantum and classical layers.
Despite these challenges, the research overall highlights the ability of even simple quantum circuits to enhance traditional neural networks, particularly in complex EO tasks such as image classification. As quantum hardware continues to improve, the practical deployment of hybrid models could lead to more advances in EO by improving the efficiency and accuracy of data processing at scale. The study also points to promising directions for further research, such as optimizing quantum architectures, refining initialization techniques to minimize variability, and expanding the use of quantum-enhanced models to more complex and diverse EO datasets.
Contributing authors on the study include Lorenzo Papa, Alessandro Sebastianelli, Gabriele Meoni, and Irene Amerini.