Quantum-Enhanced Model Offers Hope For Breast Cancer Imaging Improvements
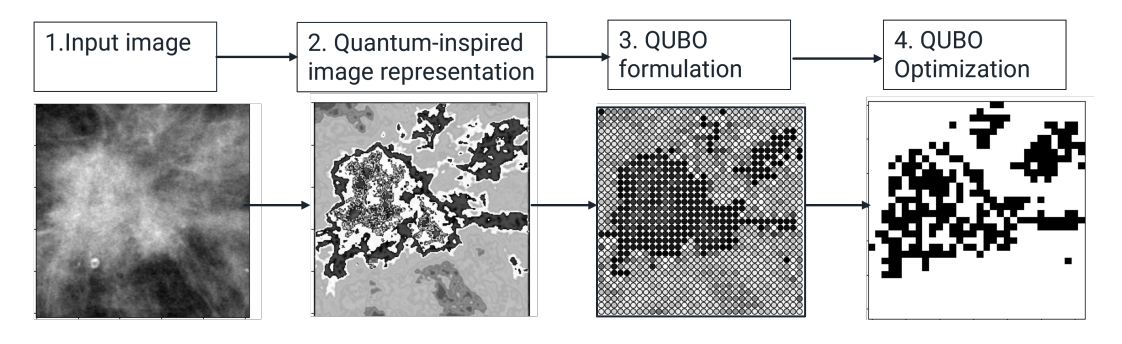
Insider Brief
- Researchers at Ingenii Inc have developed a quantum-enhanced framework for unsupervised breast cancer image segmentation that achieves accuracy comparable to state-of-the-art methods while significantly reducing computational time.
- The framework leverages quantum annealing and other quantum-inspired techniques to process images up to ten times faster than classical methods without requiring annotated datasets.
- This work could lead to processes that streamline cancer diagnostics, lower costs and improve access to advanced imaging in resource-limited settings.
A team of researchers at Ingenii Inc has developed a quantum-enhanced framework for analyzing mammography images, offering a more efficient and accurate alternative to traditional methods. This innovative approach could improve breast cancer detection while reducing the cost and time associated with manual review by radiologists.
The new method, published in the pre-print server ArXiv, uses quantum computing principles to automate the segmentation of mammography images, an important step in identifying abnormal lesions. Unlike existing techniques that rely on large annotated datasets and struggle to generalize, the proposed framework operates in an unsupervised manner and achieves performance comparable to state-of-the-art supervised models. The study found that quantum annealing — a quantum optimization technique — outperformed classical methods in speed and stability, processing images up to ten times faster without compromising accuracy.
The researchers — Laia Domingo and Mahdi Chehimi — write in the paper: “Our comparative analysis revealed that quantum and quantum-inspired methods, particularly quantum annealing and VQAs, achieved performance on par with classical optimization techniques like Gurobi, and even approached the effectiveness of state-of-the-art supervised models such as U-Net and ResUNet. Remarkably, these quantum methods operated in an
unsupervised manner, yet they managed to provide a high-fidelity approximation of the true segmentation masks, demonstrating their potential as robust alternatives when labeled data is scarce or unavailable.”
Saving Time, Saving Lives?
This development holds promise for addressing critical challenges in breast cancer diagnostics. By reducing reliance on annotated datasets, the framework lowers barriers for implementation in under-resourced medical settings.
The time savings achieved through quantum annealing could expedite diagnosis and treatment, and, especially in cancer diagnosis where speed and accuracy are vital, the method could potentially improve patient outcomes. The researchers envision future applications extending to other imaging modalities, such as 3D mammography or MRI scans, broadening the scope of quantum-enhanced medical imaging.
Methods
The team’s approach begins with a quantum-inspired transformation of mammography images to highlight regions of interest. These images are then analyzed using a process known as Quadratic Unconstrained Binary Optimization (QUBO), which formulates the segmentation task as a problem of maximizing contrast between tumor regions and the background while ensuring cohesive segmentation. The framework integrates quantum annealing and variational quantum circuits, leveraging their computational power to produce results similar to supervised deep learning models like U-Net without requiring labeled datasets.
The study benchmarked quantum methods against classical alternatives, including supervised and unsupervised models. Results showed that quantum-enhanced approaches closely matched the accuracy of supervised models while significantly outperforming unsupervised classical methods like Otsu thresholding. For example, the quantum annealing model achieved a Dice score—an accuracy metric—comparable to U-Net, a leading neural network model, despite requiring no prior training. Execution times for quantum annealing were an order of magnitude shorter than those of classical optimization software, demonstrating its efficiency.
Limitations and Future Directions
There is still work to do, according to the team. The framework’s current implementation is limited to low-resolution images (42 × 42 pixels), a necessary constraint for compatibility with available quantum hardware. Variational quantum circuits, another component of the study, also require extensive computational resources, making their practicality reliant on advances in quantum technology.
Future research could focus on scaling the framework to higher resolutions and exploring more complex imaging modalities. The researchers also aim to integrate tensor network-based methods to handle high-dimensional data efficiently and to deploy their algorithms on emerging quantum hardware to further reduce computational demands.
Quantum in Future Healthcare
This study shows how scientists in the future could apply quantum computing to healthcare. The unique ability of quantum algorithms to solve optimization problems rapidly positions them as important technological partners in medical imaging. While the field remains in its infancy, the team has demonstrated that quantum-inspired techniques can complement existing technologies, paving the way for their eventual integration into clinical practice.
As quantum hardware continues to mature, applications should develop alongside the advance.
ArXiv is a pre-print server, which means the study has yet to be officially peer-reviews. Scientists often use pre-print servers to gain informal peer feedback. The paper is highly technical and can provide readers with more of a deeper dive into the science and technology than this summary article can offer. Please read the paper here.