NVIDIA cuQuantum 23.10: Accelerating Quantum Computing with Enhanced SDK
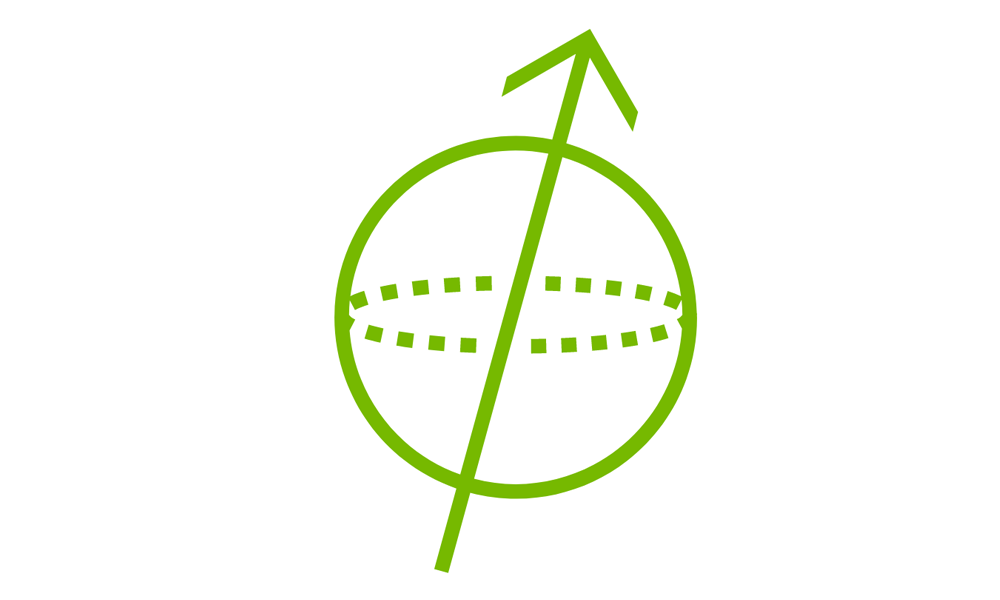
Insider Brief
- NVIDIA offered information on its latest update to its cuQuantum software development kit (SDK).
- The toolkit ntegrates more seamlessly with NVIDIA Tensor Core GPUs, greatly enhancing the speed of quantum circuit simulations.
- cuQuantum is able to accelerate quantum circuit simulations using state vector and tensor network method.
NVIDIA’s latest update to its cuQuantum software development kit (SDK), version 23.10, marks a significant bump in quantum computing capabilities, according to the company’s technical blog.
This toolkit, designed to optimize libraries and tools for quantum computing workflows, now integrates more seamlessly with NVIDIA Tensor Core GPUs, greatly enhancing the speed of quantum circuit simulations.
The core of cuQuantum’s power lies in its ability to accelerate quantum circuit simulations using state vector and tensor network methods. This advancement is not just incremental but is measured in orders of magnitude, offering unprecedented speed and efficiency in quantum computing tasks.
Key highlights of the cuQuantum 23.10 update include significant improvements to NVIDIA’s cuTensorNet and cuStateVec. The new version supports NVIDIA Grace Hopper systems, expanding the range of hardware compatible with cuQuantum. This compatibility ensures that users can leverage the full power of GPU acceleration for their quantum computing workloads.
cuTensorNet, a component of cuQuantum, now offers high-level APIs that simplify quantum simulator development. These APIs allow developers to program intuitively, abstracting complex tensor network knowledge. This simplification is crucial for building tensor-network-based quantum simulators, covering various elements like expectations, measurements, and samples. Performance-wise, cuTensorNet has shown to outperform existing technologies like TensorCircuit, PyTorch, and JAX by a factor of 4-5.9x on NVIDIA H100 GPUs.
The update also introduces experimental support for gradient calculations in quantum machine learning (QML) applications. This feature is set to accelerate QML and adjoint differentiation-based workflows significantly, utilizing cuTensorNet.
Another remarkable advancement is in cuStateVec, which now offers new APIs for host-to-device state vector swap. This development means that CPU memory can be utilized alongside GPUs to scale simulations more effectively. For example, 40 qubit state vector simulations, which previously required 128 NVIDIA H100 80GB GPUs, can now be achieved with just 16 NVIDIA Grace Hopper systems. This reduction not only speeds up computations but also leads to considerable cost and energy savings.
cuQuantum 23.10 also has undergone additional API-level and kernel-level optimizations to boost performance. These improvements make Grace Hopper systems more efficient than other CPU and Hopper systems, offering faster runtimes due to enhanced chip-to-chip interconnects and CPU capabilities.
For those interested in exploring cuQuantum 23.10, NVIDIA provides comprehensive documentation and benchmark suites on GitHub. The company encourages feedback and queries through its GitHub platform, ensuring continuous improvement and support for its user base. With these updates, NVIDIA continues to push the boundaries of quantum computing, making it more accessible and efficient for a broader range of applications.
Here’s a link to help get started.