New Study Reveals How Deep Learning Aids Quantum Field Simulation
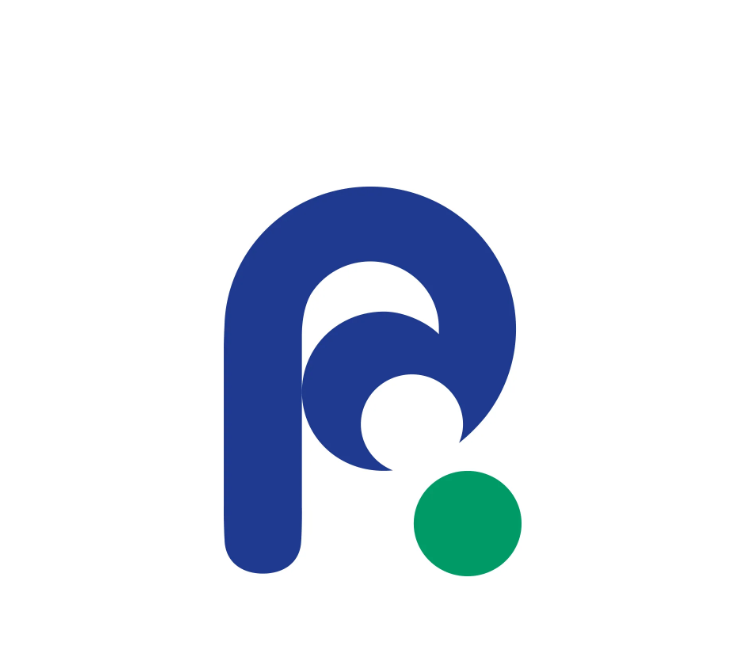
Insider Brief
- RIKEN researchers found that deep learning techniques, specifically generative diffusion, can speed up solutions to complex quantum problems by paralleling quantum simulation methods.
- They demonstrated that stochastic quantization, a quantum field theory method, is closely related to generative diffusion in deep learning, enabling faster lattice field theory simulations.
- The team plans to use this approach to tackle more complex quantum systems in future research.
A team led by RIKEN researcher Lingxiao Wang has found that mathematical solutions to complex quantum problems can be achieved more quickly by leveraging similarities between deep learning and quantum simulations.
In a study published in the Journal of High Energy Physics, the researchers discovered that stochastic quantization, a method used in quantum field theory, closely parallels the statistical technique of generative diffusion used in deep learning.
“We’ve shown that models based on generative diffusion provide a powerful framework for exploring quantum field theories on a lattice,” said Wang.
By applying this insight to lattice field theory simulations, they were able to find solutions much faster than before. The team plans to use this approach to explore more complex systems in the future.
Wang explained that their research demonstrated how models based on generative diffusion offer a powerful framework for exploring quantum field theories on a lattice. He noted that stochastic quantization, which involves introducing quantum noise into the field system, allows for a probabilistic interpretation of quantum fields. According to Wang, this approach is particularly useful in lattice field theory as it provides an alternative method for simulating quantum field theories on a computer.