‘Nanomagnetic’ Computing Could Drastically Cut AI’s Energy Use
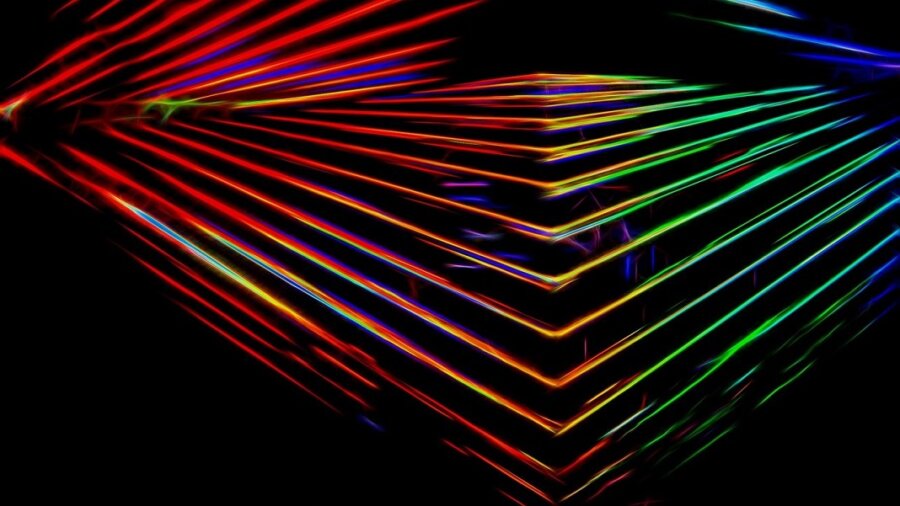
As the Internet of Things expands, engineers want to embed AI into everything, but the amount of energy it requires is a challenge for the smallest and most remote devices. A new “nanomagnetic” computing approach could provide a solution.
While most AI development today is focused on large, complex models running in huge data centers, there is also growing demand for ways to run simpler AI applications on smaller and more power-constrained devices.
For many applications, from wearables to smart industrial sensors to drones, sending data to cloud-based AI systems doesn’t make sense. That can be due to concerns about sharing private data, or the inevitable delays that come from transmitting the data and waiting for a response.
But many of these devices are too small to house the kind of high-powered processors normally used for AI. They also tend to run on batteries or energy harvested from the environment, and so can’t meet the demanding power requirements of conventional deep learning approaches.
This has led to a growing body of research into new hardware and computing approaches that make it possible to run AI on these kinds of systems. Much of this work has sought to borrow from the brain, which is capable of incredible feats of computing while using the same amount of power as a light bulb. These include neuromorphic chips that mimic the wiring of the brain and processors built from memristors—electronic components that behave like biological neurons.
New research led by scientists from Imperial College London suggests that computing with networks of nanoscale magnets could be a promising alternative. In a paper published last week in Nature Nanotechnology, the team showed that by applying magnetic fields to an array of tiny magnetic elements, they could train the system to process complex data and provide predictions using a fraction of the power of a normal computer.
At the heart of their approach is what is known as a metamaterial, a man-made material whose internal physical structure is carefully engineered to give it unusual properties not normally found in nature. In particular, the team created an “artificial spin system,” an arrangement of many nanomagnets that combine to exhibit exotic magnetic behavior.
Their design is made up of a lattice of hundreds of 600-nanometer-long bars of permalloy, a highly magnetic nickel-iron alloy. These bars are arranged in a repeating pattern of Xs whose upper arms are thicker than their lower arms.
Normally artificial spin systems have a single magnetic texture, which describes the pattern of magnetization across its nanomagnets. But the Imperial team’s metamaterial features two distinct textures and the ability for different parts of it to switch between them in response to magnetic fields.
The researchers used these properties to implement a form of AI known as reservoir computing. Unlike deep learning, in which a neural network rewires its connections as it trains on a task, this approach feeds data into a network whose connections are all fixed and simply trains a single output layer to interpret what comes out of this network.
It’s also possible to replace this fixed network with physical systems, including things like memristors or oscillators, as long as they have certain properties, such as a non-linear response to inputs and some form of memory of previous inputs. The new artificial spin system fits those requirements, so the team used it as a reservoir to carry out a series of data-processing tasks.
They input data to the system by subjecting it to sequences of magnetic fields before allowing its own internal dynamics to process the data. They then used an imaging technique called ferromagnetic resonance to determine the final distribution of the nanomagnets, which provided the answer.
While these were not practical data-processing tasks, the team was able to show that their device was able to match leading reservoir computing schemes on a series of prediction challenges involving data that varies over time. Importantly, they showed that it was able to learn efficiently on fairly short training sets, which would be important in many real-world IoT applications.
And not only is the device very small, the fact that it uses magnetic fields to carry out computation rather than shuttling electricity around means it consumes far less power. In a press release, the researchers estimate that when scaled up it could be 100,000 times more efficient than conventional computing.
There’s a long way to go before this kind of device could be put to practical use, but the results suggest computers based on magnets could play an important role in embedding AI everywhere.
Image Credit: BarbaraJackson / 264 images