Moderna, IBM Quantum Researchers Use Quantum Computers For Critical Step in RNA-based Therapeutic Design
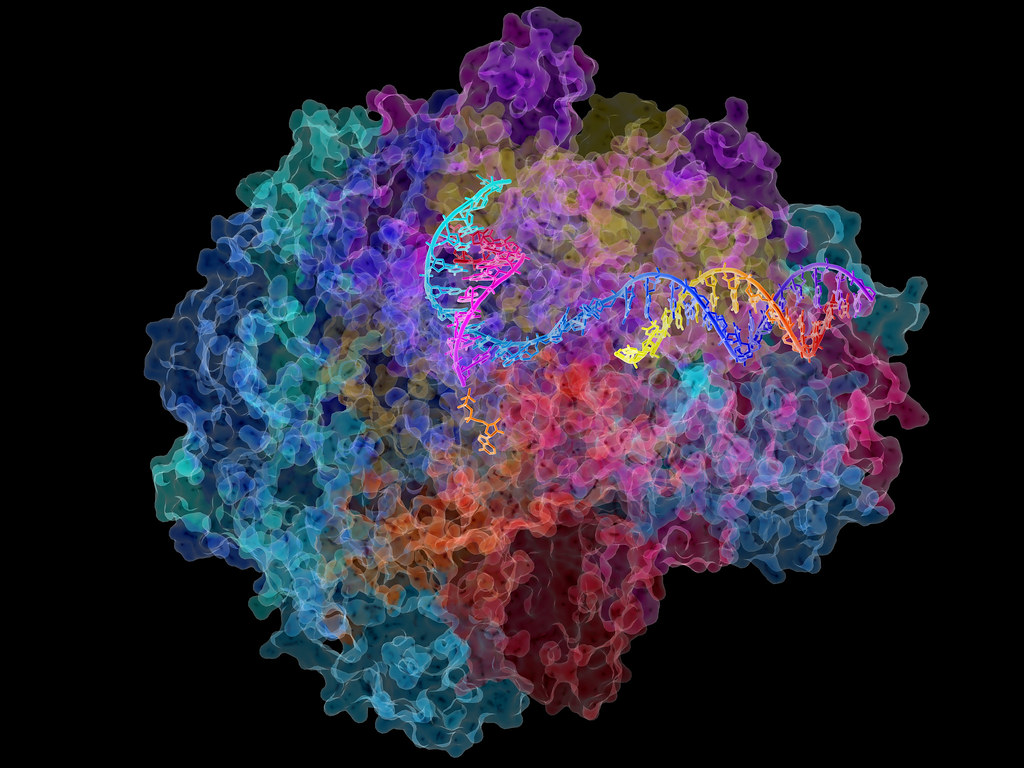
Insider Brief
- Moderna and IBM Quantum researchers have successfully used quantum computers predict mRNA secondary structures.
- The effort shows the promise of quantum computers to enhance mRNA research, specifically, as well as marking a significant step in computational biology.
- Because the team derived results with relatively basic error correction techniques, the study suggests that quantum computing is approaching a utility-scale capability for solving real-world problems.
Moderna and IBM Quantum researchers have successfully used quantum computers predict mRNA secondary structures, a critical aspect of designing RNA-based therapeutics, according to a paper posted on the pre-print server ArXiv.
The team writes that this collaborative effort shows the promise of quantum computers to enhance mRNA research, specifically, as well as marking a significant step in computational biology. IBM and Moderna announced the research collaboration last year.
Messenger RNA (mRNA) serves as an intermediary between DNA and proteins, playing a pivotal role in gene expression. The secondary structure of mRNA, which includes intricate folding patterns due to intramolecular base pairing, is crucial for its functionality. Accurate prediction of these structures is essential for understanding gene regulation, translation, and degradation, and for designing effective RNA-based treatments.
Traditionally, predicting mRNA secondary structures has been a challenging task for classical computers due to the complexity of the problem. Quantum computers, however, offer a new approach. Because quantum computing technology has grown increasingly more advanced, the researchers were able to tackle this complex problem more efficiently.
In their study, the researchers used the IBM Eagle and Heron quantum processors to solve mRNA structure prediction problems. They employed the Conditional Value at Risk (CVaR)-based Variational Quantum Eigensolver (VQE) algorithm, which is designed to handle optimization problems. This algorithm was used to predict the minimum free energy (MFE) structures of mRNA sequences, a key factor in determining the stability and functionality of the mRNA.
The study focused on mRNA sequences up to 60 nucleotides in length, representing problems within the range of 10 to 80 qubits. Despite the inherent noise and errors in current quantum hardware, the researchers achieved accurate predictions that matched the results from classical solvers like CPLEX. This success was achieved even with minimal error mitigation and relatively shallow quantum circuits, a key goal in the investigation.
They write: “The goal here is to demonstrate that variational quantum algorithms can be fully and successfully executed on such hardware, with hundreds of quantum circuit calls during the hyperparameter training step along with basic error mitigation, yielding accurate results that match those obtained with classical optimization schemes.”
The team conducted noise-free simulations using the Matrix-Product State (MPS) simulator in Qiskit, IBM’s quantum computing software development framework. The simulations were limited to shallow-depth circuits due to the exponential growth of computational cost with entanglement. They tested different values of the CVaR parameter and found that a setting of 0.1 provided reliable results across various problem sizes. Each simulation run involved multiple independent trials to ensure the robustness of the optimization process.
One of the more significant findings was that even noisy quantum hardware could produce reliable results for optimization-based problems of relatively large scales. This suggests that quantum computing is approaching a utility-scale capability for solving real-world problems. The study also highlights the potential of low-overhead error mitigation schemes to enhance the accuracy of quantum computations.
The implications of this research are significant for the field of bioinformatics, according to the researchers. Quantum computing could provide valuable insights into RNA folding mechanisms, enabling the rapid identification of functional RNA structures. This is particularly important for the development of RNA-based therapeutics, such as mRNA vaccines, which have gained prominence in recent years due to their role in combating diseases like COVID-19.
The researchers acknowledge that the scalability of their methods to problem sizes exceeding 100 qubits remains an open question. Although it’s a promising step toward quantum advantage — the point where a quantum computer can solve a problem more efficiently than the best-known classical computers — it may be a bit premature to say this study is an example of quantum advantage.
However, the researchers point out that although this work might be just the beginning of investigations into how quantum computing can tackle bioinformatic tasks that are too complex for classical computers, it’s important foundational work. Future work will focus on exploring more advanced optimization techniques and further improving the capabilities of quantum hardware. As quantum technology continues to advance, it is expected that these improvements will bolster the prospects of using quantum computers for complex biological problems.
“Our results indicate that quantum computing offers valuable insights into RNA folding mechanisms and enables rapid
identification of functional RNA structures,” the scientists write. “This work lays the groundwork for future research in quantum-assisted bioinformatics and highlights the importance of developing scalable quantum algorithms for real-world biological problems.”
Although COVID vaccines might be the most immediate and well known example of mRNA therapeutics, researchers suggest that mRNA technology holds promise for a wide range of future medical treatments. It could be used to develop vaccines for other infectious diseases, such as influenza and HIV, for example. Other research teams are looking into whether mRNA can be employed in cancer immunotherapy to prompt the immune system to target and destroy cancer cells. It may also be used for protein replacement therapies to treat genetic disorders by instructing cells to produce missing or defective proteins, offering potential treatments for diseases like cystic fibrosis and muscular dystrophy.
The research team included Dimitris Alevras, Vaibhaw Kumar, Triet Friedhoff, Jae-Eun Park, and Mariana LaDue from IBM Quantum in New York, USA; Takahiro Yamamoto and Mitsuharu Takeori from IBM Quantum in Tokyo, Japan; and Mihir Metkar, Wade Davis, and Alexey Galda from Moderna in Cambridge, USA.