Kipu Quantum Team Says New Quantum Algorithm Outshines Existing Techniques
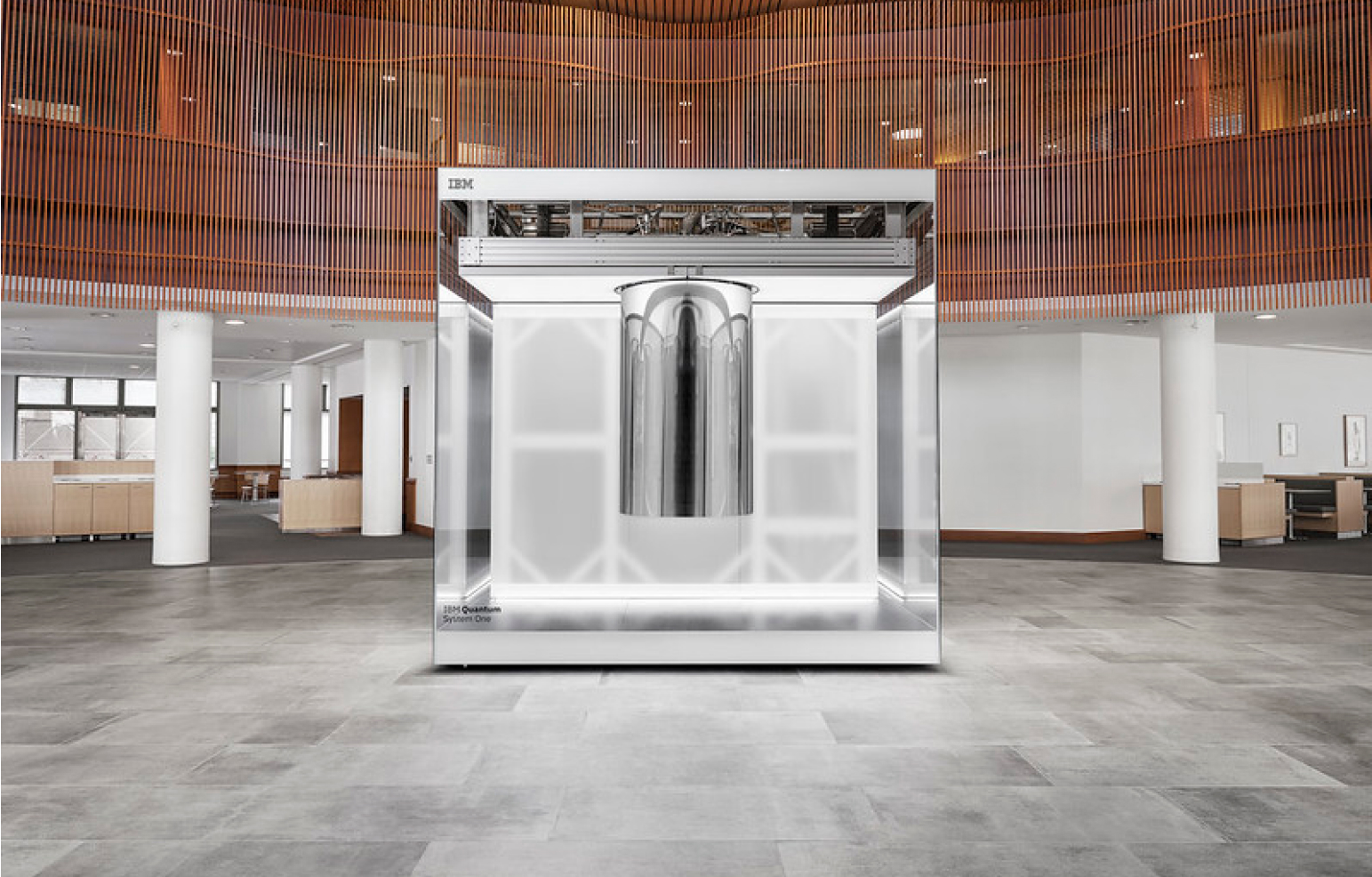
Insider Brief
- Kipu Quantum’s team successfully tested the largest quantum optimization problem on a 156-qubit IBM quantum processor using their BF-DCQO algorithm, surpassing existing quantum and classical optimization methods.
- The BF-DCQO algorithm, designed for higher-order unconstrained binary optimization (HUBO) problems, outperformed the Quantum Approximate Optimization Algorithm (QAOA), quantum annealing, and classical techniques like Tabu search.
- Kipu Quantum also simulated the algorithm on a 433-qubit IBM Osprey processor, suggesting that the method can scale effectively, potentially marking the start of the commercial quantum advantage era.
- Image: IBM Quantum System One
installed at Cleveland Clinic.
Kipu Quantum-led team of researchers announced the successful testing of what they’re labeling the largest quantum optimization problem on a digital quantum computer. They suggest that this is the start of the commercial quantum advantage era.
“Today, we are proud to present the largest optimization quantum algorithm via an experiment running on an IBM quantum processor using all 156 qubits by applying Kipu’s powerful algorithms,” the Kipu Quantum team writes in a LinkedIn post. “This is just the beginning, as our methods can be directly applied on larger processors, including the one with 433 qubits and beyond, which brings about a fundamental change in the perspective of current quantum computing possibilities.”
In a paper, the team reports that their algorithm, designed to address higher-order unconstrained binary optimization (HUBO) problems, has shown improved performance over existing methods in both experimental and simulated tests.
The new bias-field digitized counterdiabatic quantum optimization (BF-DCQO) algorithm was tested using 156 qubits on IBM’s quantum processor with a heavy-hex architecture, a hexagon-shaped pattern designed to reduce errors in quantum gates and enhances qubit connectivity.
The results surpassed the performance of standard quantum optimization methods, including the quantum approximate optimization algorithm (QAOA), quantum annealing, simulated annealing, and classical approaches like Tabu search. Additionally, Kipu Quantum simulated a similar HUBO problem on IBM’s upcoming 433-qubit Osprey processor, providing evidence that the method could scale effectively on larger quantum systems.
“We consider these results of our case studies as the start of the Commercial Quantum Advantage era, Kipu dixit, and soon more and better when our technologies are extended to denser and more complex industry-level problems,” the team writes in the post.
Real-World Uses
Combinatorial optimization is critical in many industries, from logistics and scheduling to computational chemistry and biology. These problems, which involve finding the best or near-optimal solutions in large discrete configuration spaces, are known to be computationally challenging, particularly for classical computing. This complexity has driven the exploration of quantum optimization techniques as an alternative.
Kipu Quantum’s BF-DCQO algorithm leverages quantum computing’s potential to solve such combinatorial optimization problems, specifically by using the Ising spin-glass model to encode the HUBO problems. The BF-DCQO algorithm incorporates an enhanced bias term that improves the performance of quantum systems in tackling these complex problems. This new protocol has been experimentally validated, showing promise for addressing more industry-level HUBO problems in the near future.
Two-Stage Test
Kipu Quantum’s BF-DCQO algorithm was tested in two stages: first on IBM’s 156-qubit quantum processor and then through a simulation of the upcoming 433-qubit Osprey processor. In both instances, the algorithm was compared to established quantum and classical optimization methods. The company applied matrix product state (MPS) simulation techniques to evaluate the feasibility of the HUBO problem on the larger 433-qubit processor.
The BF-DCQO algorithm’s advantage lies in its ability to efficiently handle complex optimization problems with up to three-body interactions in the nearest-neighbor Ising spin-glass model. This capability makes it particularly well-suited for heavy-hex qubit architectures like those used in IBM’s quantum processors.
The study also extended the method to tackle a weighted MAX 3-SAT problem, a binary satisfiability problem often used as a benchmark in combinatorial optimization. The results demonstrated that BF-DCQO could outperform classical algorithms and quantum methods like those offered by D-Wave, which naturally handle quadratic unconstrained binary optimization (QUBO) problems.
Limitations of the Study
While Kipu Quantum’s BF-DCQO algorithm shows promise, the results are based on simulations and experiments using specific quantum architectures. The 156-qubit experimental validation was performed on IBM’s heavy-hex processor, while the 433-qubit simulation is yet to be fully realized on physical hardware. There are still challenges in scaling the method to address more complex real-world HUBO problems that require larger quantum systems.
It should also be noted that BF-DCQO algorithm has so far been tested on relatively idealized conditions, with simulations being noise-free. As quantum computing hardware advances, real-world noise and error correction issues will need to be addressed to maintain the algorithm’s effectiveness.
Likely all of the above will be the focus of upcoming investigations.
Future Research Directions
Kipu Quantum plans to extend its BF-DCQO algorithm to tackle more industry-related HUBO problems, including applications in fields like logistics, computational biology, and chemistry. As quantum hardware continues to evolve, the company aims to validate its findings on larger quantum processors, particularly IBM’s Osprey processor with 433 qubits.
Future research will likely explore how the BF-DCQO algorithm performs in noisier quantum environments and how it can be optimized for larger and denser HUBO instances. Kipu Quantum also hopes to integrate the algorithm into commercial quantum computing platforms, which could mark a step toward the practical application of quantum advantage in solving combinatorial optimization problems.
The research on the Bias-Field Digitized Counterdiabatic Quantum Algorithm for Higher-Order Binary Optimization is led by several researchers, including: Sebastián V. Romero and Alejandro Gomez Cadavid are affiliated with both Kipu Quantum GmbH in Berlin, Germany, and the University of the Basque Country UPV/EHU in Bilbao, Spain. Anne-Maria Visuri and Enrique Solano are also based at Kipu Quantum GmbH, while Narendra N. Hegade is another key contributor from the same institution in Berlin.