In the Fight Against Noisy Quantum Computing, CVaR Proves a Worthy Opponent
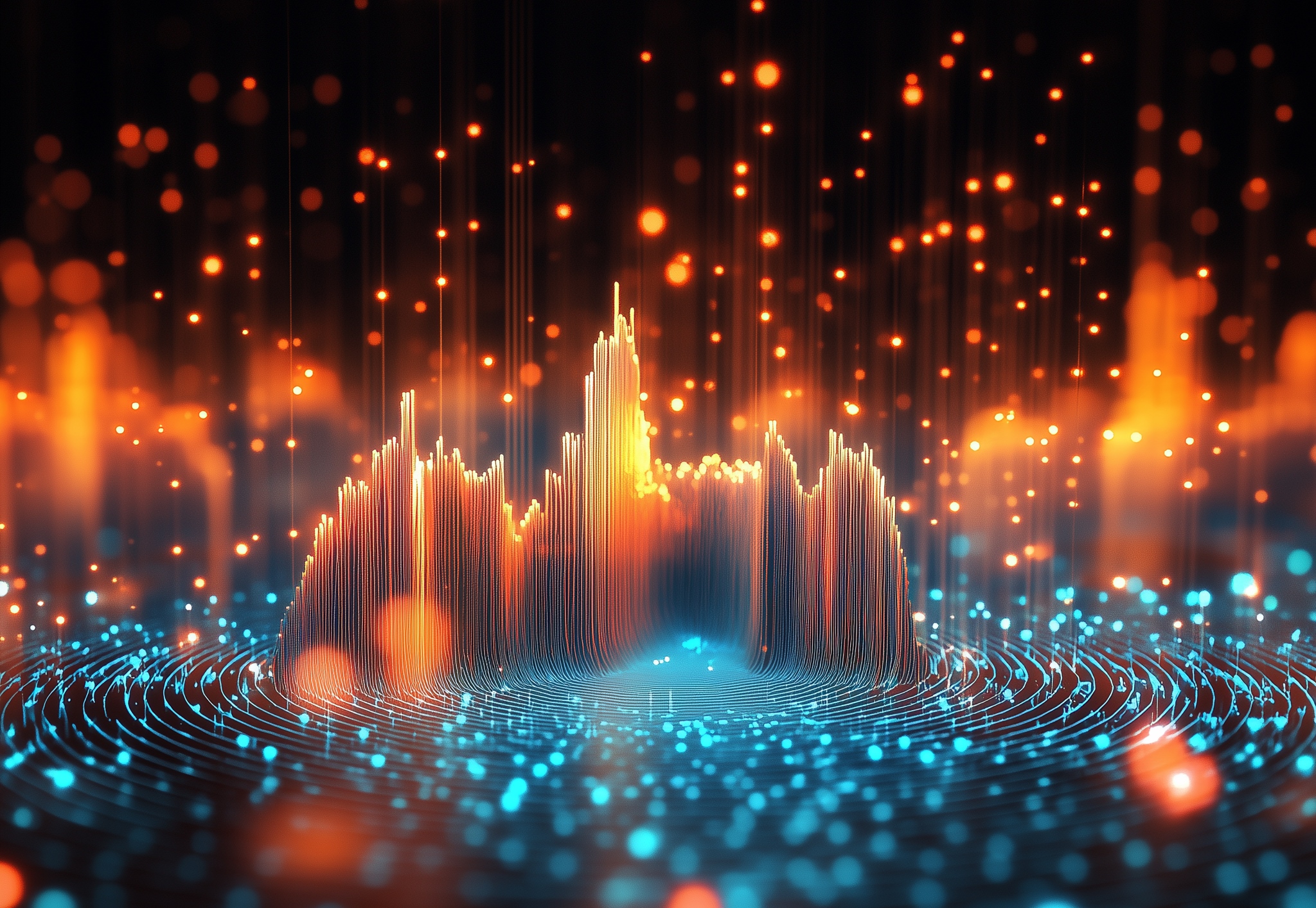
Insider Brief:
- Quantum computing’s potential is limited by noise, which affects the accuracy of data output, prompting researchers to explore new noise management methods.
- IBM Quantum and Los Alamos National Laboratory proposed using Conditional Value at Risk (CVaR), a tool from finance, to provide accurate bounds on noise-free results in quantum systems.
- By focusing on worst-case outcomes, CVaR enables reliable fidelity estimation between quantum states, which is essential for algorithms in quantum machine learning and optimization.
- This CVaR approach could reduce sampling demands as compared to traditional error mitigation methods, providing a scalable solution for noise management as quantum computing evolves.
Quantum computing, though oft found in discussions speaking to its (potential) exponential computational power and (possibly one day) ability to solve our hardest problems, is not fairly represented without mentions of its most profound foe: noise. Current quantum computers are inherently noisy, and this noise can cloud the accuracy of the data they produce. In a recently published research paper Nature Computational Science, a team from IBM Quantum and Los Alamos National Laboratory presented a new approach to managing noise through conditional value at risk (CVaR), which could lead to more accurate calculations on near-term quantum devices.
The Undeniable Noise Challenge
While today’s quantum devices have undeniably progressed in a trajectory toward practical application, they are equally undeniably limited by high levels of noise that compromise the accuracy of results. As noted in the study, the presence of noise in quantum systems means that extracting useful samples—essentially accurate representations of the quantum states—is a daunting challenge.
Researchers have traditionally used error mitigation techniques such as probabilistic error cancellation and zero-noise extrapolation to manage this issue. However, these methods often come at a steep cost in terms of computational resources. Notably, error mitigation scales exponentially, making it impractical for larger quantum systems due to the substantial increase in required samples.
Instead, the researchers explored using the conditional value at risk as a less resource-intensive method. CVaR, often used in finance, is a measure that estimates the risk of extreme losses by focusing on the worst-case outcomes within a certain probability range. By applying CVaR to noisy samples, they demonstrated that it’s possible to achieve provable bounds on “noise-free” expectation values, providing a solid framework for handling noise without the prohibitive sampling overhead typical of PEC and ZNE.
CVaR and Its Role in Fidelity Estimation
The CVaR method has distinct advantages over traditional noise mitigation. As a statistical measure commonly used in finance to evaluate the tail risk—essentially the likelihood of extreme outcomes—the researchers found that this same principle could be applied to noisy quantum samples to predict noise-free results.
By focusing on the “upper quantiles” of sample data, CVaR allowed the researchers to establish reliable bounds, which, as the study shows, provides an efficient means of estimating fidelities between quantum states. This fidelity estimation is essential for algorithms that rely on consistent measurements between quantum states, such as quantum support vector machines and variational quantum time evolution, both widely used in quantum machine learning and optimization.
As demonstrated in the study, the CVaR approach required significantly fewer samples than PEC. According to the team, this reduced overhead makes CVaR a promising tool for tasks that prioritize accuracy over a full correction, such as fidelity estimation, where researchers seek to understand how closely a noisy quantum state mirrors a theoretical, noise-free state.
Quantum Optimization and Conditional Value at Risk
When considering the areas we expect to see quantum advantage, optimization is often includes as one of the top categories and has broad applications across industries from supply chain optimization, transport routing, task scheduling, and more. In quantum optimization algorithms, such as the quantum approximate optimization algorithm, each output bit string from the quantum computer represents a potential solution to the problem at hand. As highlighted in the paper, obtaining accurate samples is necessary for success in these optimization tasks, yet noise often makes this challenging. The team found that CVaR could be used to achieve performance guarantees similar to what would be expected on noise-free hardware.
In experiments conducted on real quantum devices, including IBM’s 127-qubit systems, CVaR allowed researchers to confidently address noise while minimizing the extra sample count required. This is especially valuable in tasks like the MaxCut problem, where performance metrics on noisy hardware need to match theoretical expectations. “It turns out that the sampling overhead is substantially lower than, for example, estimating expectation values via PEC,” the team explained, emphasizing that CVaR effectively reduces the sampling burden while preserving result quality.
Applications and Implications for Quantum Computing
Beyond the immediate technical improvements, the results of this study are relevant across quantum fields. Fidelity estimation, as discussed, is essential for machine learning tasks where the accuracy of quantum state mappings directly impacts algorithm performance. Additionally, CVaR’s noise-handling capabilities are expected to support more accurate modeling in quantum optimization, providing new opportunities in fields that range from logistics and finance to complex scientific modeling.
The study concludes that CVaR could serve as a practical approach to noise management in the near-term, where quantum error correction is not yet fully realized. As quantum hardware continues to evolve, the approach outlined here will likely remain relevant.
Contributing authors on the study include Samantha V. Barron, Daniel J. Egger, Elijah Pelofske, Andreas Bärtschi, Stephan Eidenbenz, Matthis Lehmkuehler, and Stefan Woerner.