Deloitte Italy Explores Quantum Machine Learning for Digital Payments Fraud Detection
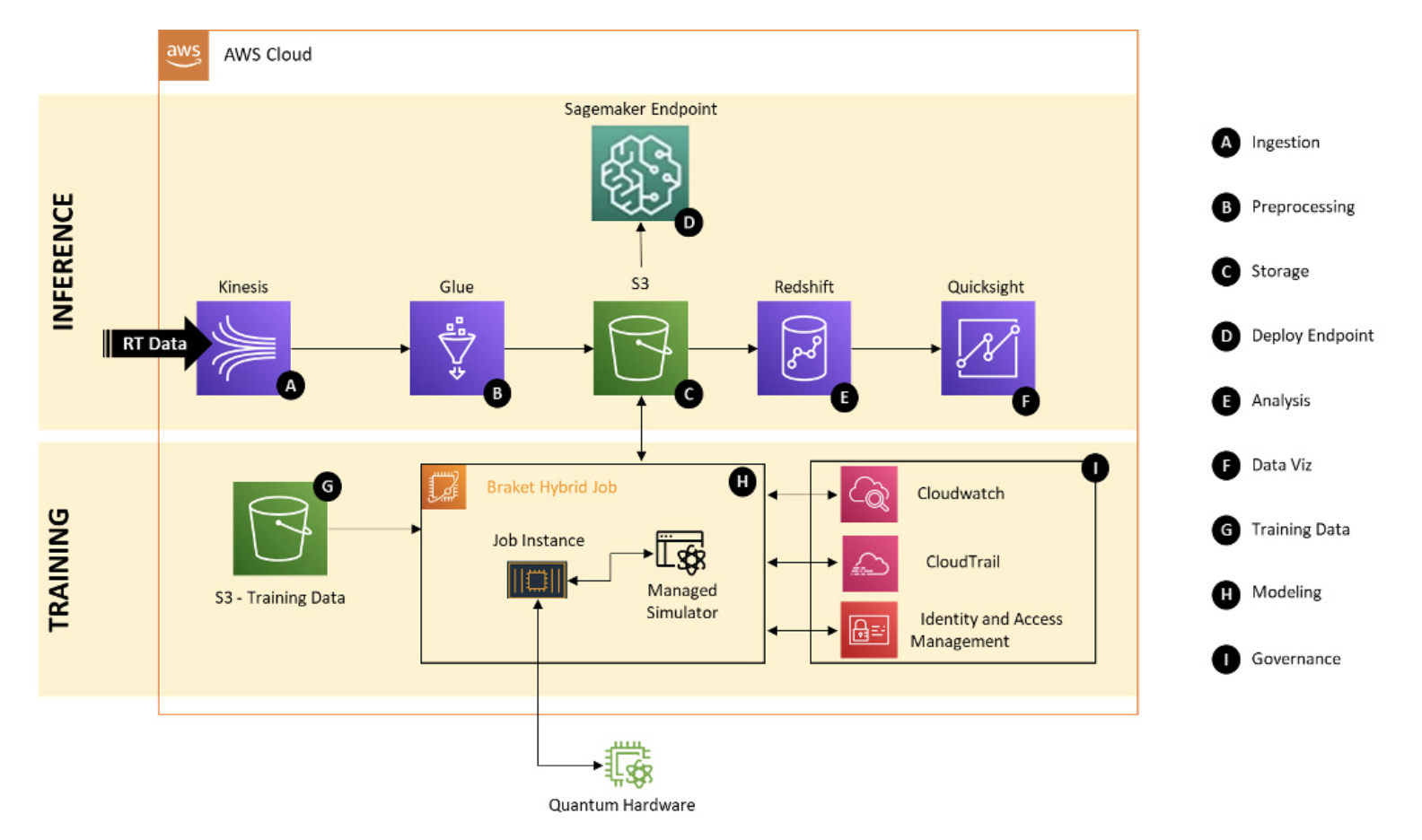
Insider Brief
- Deloitte Italy partnered with Amazon Braket to investigate the power of quantum machine learning (QML) to enhance the detection of fraudulent transaction.
- Deloitte’s quantum-enhanced fraud detection system uses a hybrid quantum neural network.
- The hybrid approach leverages the strengths of both classical and quantum computing.
Deloitte Italy is reporting on their partnership with Amazon Braket, Amazon’s quantum computing service, to investigate the power of quantum machine learning (QML) in enhancing the detection of fraudulent transactions — and the exploration may serve as a glimpse into the future of financial security.
According to the team, writing in the AWS Machine Learning Blog, as e-commerce continues its explosive growth, the need for robust and adaptable fraud detection mechanisms are more and more critical. Traditional machine learning (ML) algorithms have been instrumental in analyzing vast amounts of transactional data in real time, swiftly identifying suspicious activities. However, integrating quantum computing may help push these capabilities even further.
Investing in Quantum
Quantum computing, still in its nascent stage, has the potential to transform computation in various sectors, including finance, the team writes.
Unlike classical computers, which use bits as the smallest unit of information, quantum computers use qubits. These qubits exploit quantum phenomena like superposition and entanglement, allowing them to theoretically process complex calculations at unprecedented speeds. This makes them particularly well-suited for optimization problems and simulations that are infeasible for traditional computers. Those types of calculations are important for solving many of challenges facing the financial industry today.
The team writes that when approaching quantum, it’s a marathon, not a sprint.
They report “Quantum computers harbor the potential to radically overhaul financial systems, enabling much faster and more precise solutions. Compared to classical computers, quantum computers are expected in the long run to have to advantages in the areas of simulation, optimization, and ML. Whether quantum computers can provide a meaningful speedup to ML is an active topic of research.”
Deloitte’s quantum-enhanced fraud detection system employs a hybrid quantum neural network. The neural network, built using the Keras library, incorporates a quantum component implemented with PennyLane, a software framework for quantum ML. This hybrid approach leverages the strengths of both classical and quantum computing, offering a pathway to more accurate and efficient fraud detection, according to the researchers.
Overcoming Challenges
Despite its promise, quantum computing faces significant hurdles. The research team lists a few of those hurdles.
Quantum computers are highly sensitive to external disturbances, such as temperature fluctuations, which can introduce noise and errors into calculations. Techniques like parametric compilation, which allows circuits to be compiled once and reused with fresh parameters, help mitigate some of these issues. Amazon Braket automates this process, which can increase the reliability of quantum computations.
The team adds that the inherent complexity of qubits and their probabilistic nature pose additional challenges.
The team writes: “By harnessing the principles of superposition and entanglement, qubits possess an elevated degree of complexity in their design. This intricate architecture renders the evaluation of computational capacity a formidable challenge, because the multidimensional aspects of qubits demand a more nuanced approach to assessing their computational prowess.”
Increased calculation errors are also a common issue, but ongoing research in error mitigation and suppression techniques aims to address these concerns, the team admits. By continuing to refine these methods, the hope is that the accuracy and reliability of quantum computing will improve — and, if successful, that could pave the way for broader adoption.
Future Prospects
The preliminary results from Deloitte’s QML-based fraud detection system are promising, demonstrating superior performance in identifying fraudulent transactions compared to traditional ML methods. As quantum computing technology matures, its role in transforming fraud detection and other critical financial processes will become more apparent.
To stay ahead of the curve, the team advises organizations to begin integrating quantum-ready solutions into their operations. While the timelines of when quantum will arrive vary, this preparation will better ensure a smooth transition to quantum computing once the hardware reaches commercial viability, according to the researchers.
For a deeper technical dive than this overview can provide, including details about the data sets and quantum circuits, please review the post.
A little background on the research team: Federica Marini, a manager in Deloitte Italy’s AI & Data practice, an expert in AI, Gen AI, ML, and Data, providing tailored, data-driven solutions with a human-centered approach. Matteo Capozi, also from Deloitte Italy, specializes in advanced AI, GenAI models, and quantum computing, driving innovation and strategic goals across industries. Kasi Muthu, a senior partner solutions architect at AWS in Dallas, focuses on generative AI and data, helping partners and customers accelerate their cloud journey with scalable and resilient solutions. Kuldeep Singh, a Principal Global AI/ML leader at AWS, has combined experience in deep AI, ML, and cybersecurity knowledge.