Microsoft Integrates HPC, Quantum Computing, and AI for Chemical Reactions Study
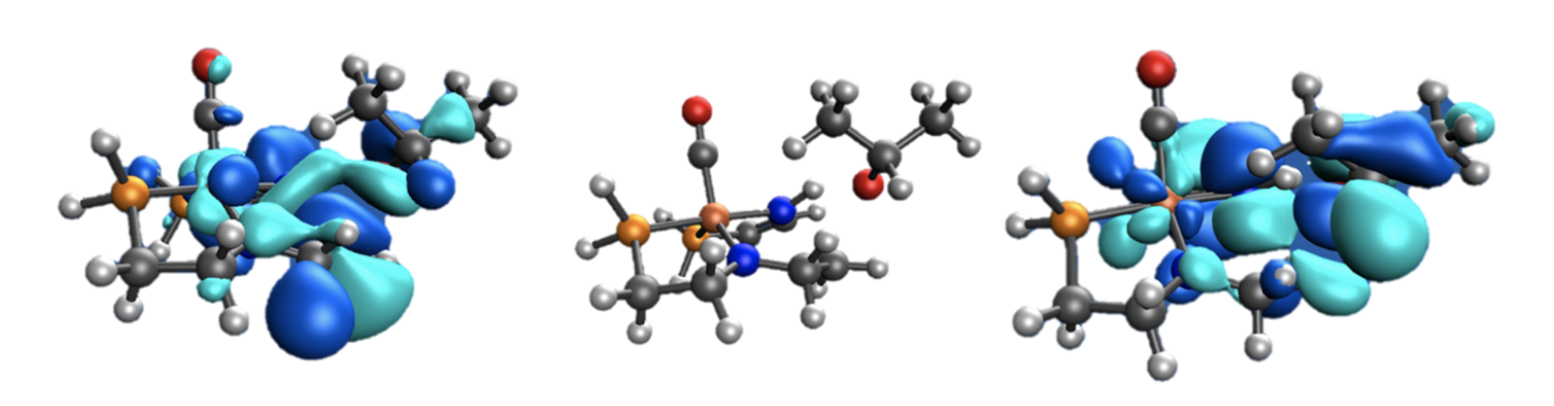
Insider Brief
- Microsoft demonstrated a case study combining HPC, quantum computing, and AI to study catalytic reactions, using logical qubits to improve the reliability of quantum simulations.
- The study involved over a million density functional theory (DFT) calculations using the Azure Quantum Elements platform to map out reaction networks, identifying over 3,000 unique molecular configurations.
- Encoded quantum computations achieved higher accuracy than unencoded ones, showing the importance of logical qubits in improving quantum calculations, though efficiency remains a challenge due to high rejection rates.
Quantum. Artificial Intelligence. High-Performance Computing.
Microsoft scientists broke out the whole computational kitchen sink as they demonstrated the integration of high-performance computing (HPC), quantum computing and artificial intelligence (AI) to study catalytic reactions that produce chiral molecules, a key area in chemistry.
And, while you’re at it, also note how the company’s researchers are using logical qubits in this study.
According to the team’s research paper on the pre-print server ArXiv, this demonstration of computational integration not only marks an important advance in the use of quantum computing for industrial and scientific applications, but, according to the researchers, can also be seen as a step forward in the combination of classical and quantum technologies. Conducted on Microsoft’s Azure Quantum Elements platform, the scientists used classical HPC simulations, quantum computing via logical qubits, and AI tools for automated reaction network analysis. The results highlight the potential of quantum computing to solve complex chemical problems that are computationally challenging for classical systems.
Azure Quantum Elements, Quantinuum Hardware
In the study, classical HPC simulations were first used to pre-process the reaction data. Microsoft employed its Azure Quantum Elements platform to run automated reaction network analyses using an AI-driven tool AutoRXN, which generated over a million density functional theory (DFT) calculations. It should be noted that generating a million density functional theory (DFT) calculations is significant because DFT is computationally intensive, as it models the quantum mechanical behavior of electrons within molecules to estimate energy states and properties. Running a million DFT calculations demonstrates the capability to explore vast chemical reaction networks in detail.
In this case, these simulations mapped out the reaction network of an iron catalyst used in producing chiral molecules, identifying over 3,000 unique molecular configurations. However, DFT approximations can introduce errors, especially in cases where quantum correlations between electrons are strong.
For more accurate results, quantum computing was used to address these highly correlated configurations. Specifically, Microsoft employed its bias-field digitized counterdiabatic quantum optimization (BF-DCQO) algorithm, which leverages logical qubits to generate the ground state of relevant electron spaces.
The quantum computation was performed on Quantinuum’s H1-1 trapped-ion quantum processor, using error-detecting codes to enhance the reliability of the quantum results.
How Quantum Computation Fits In
The quantum portion of the study centered around estimating the ground state energy of molecules, which is critical for understanding the energy barriers of chemical reactions. Using a Hamiltonian specific to the reaction’s transition states, the quantum system prepared the ground state using logical qubits encoded with a [[4, 2, 2]] error-detecting code, which means a quantum error-correcting code that uses four physical qubits to encode two logical qubits. This code allows for fault-tolerant quantum operations by detecting and rejecting errors in state preparation and measurement.
The quantum computations were complemented by classical post-processing. Randomized Pauli measurements were used to generate classical shadows of the quantum states, which were then analyzed to estimate the ground state energy. (You can read about Quantinuum’s classical shadows work here.)
The encoded quantum computations achieved chemical accuracy, with an error of 0.15 milli-Hartree, a unite used to measure energy. That computation outperformed unencoded computations, which had an error of 0.91 milli-Hartree.
One of the major findings was that the encoded quantum computations were statistically more accurate than unencoded ones. Bootstrap methods showed that with 98% probability, the encoded computation provided a closer estimate to the true ground state energy. This is important becaus4e it shows the utility of logical qubits for improving the reliability of quantum calculations. The team recognizes this as a criticL step for advancing quantum chemistry.
Limitations and Challenges
While the study shows progress, the researchers offer some limitations, as well as offer suggestions on area where more work is needed. While logical qubits improve reliability, scaling this approach to larger, more complex chemical reactions would likely present challenges. Quantum error correction increases computational overhead by requiring more qubits and measurements.
The researchers also report that the encoded quantum computations required twice as many measurement attempts (80,000 shots per setting) compared to the unencoded ones (40,000 shots per setting), but only 47% of these results were accepted after error detection, with a 3% rejection rate due to errors and an additional 50% due to teleportation flags.
While the encoded method offers higher accuracy, it points out that efficiency might be limited by the rejection rate, particularly from teleportation flags, meaning more shots are needed to obtain valid results. However, this issue can be reduced with improvements to the circuits, making it a mixed result: accurate but currently less efficient, likely another path for future research, which we’ll discuss now.
Future Research Directions
The integration of HPC, quantum computing, and AI in this study serves as a proof of concept for future large-scale quantum chemistry applications.
Further development of quantum algorithms, such as the Quantum Phase Estimation (QPE) algorithm, is also expected to improve the precision of quantum simulations. While current methods like the Variational Quantum Eigensolver (VQE) can approximate ground states, they do not scale well to larger systems. In contrast, QPE offers better scaling and guarantees chemical accuracy, making it a promising approach for future quantum chemistry applications.
Microsoft is also focusing on reducing the overhead of error correction. While logical qubits are necessary for fault tolerance, they require additional qubits and measurements, which limit the size of problems that can be addressed. Optimizing the error-detection process and developing more efficient quantum codes will be key areas of focus for future research.
Often, we can’t include some of the technological details and often a lot of the nuance is not included in these summaries, so it’s important to check out the research paper.