Researchers Say New Algorithm Outperforms Existing AI Compressors in Accuracy and Reduction of Computational Demands of GPT-2
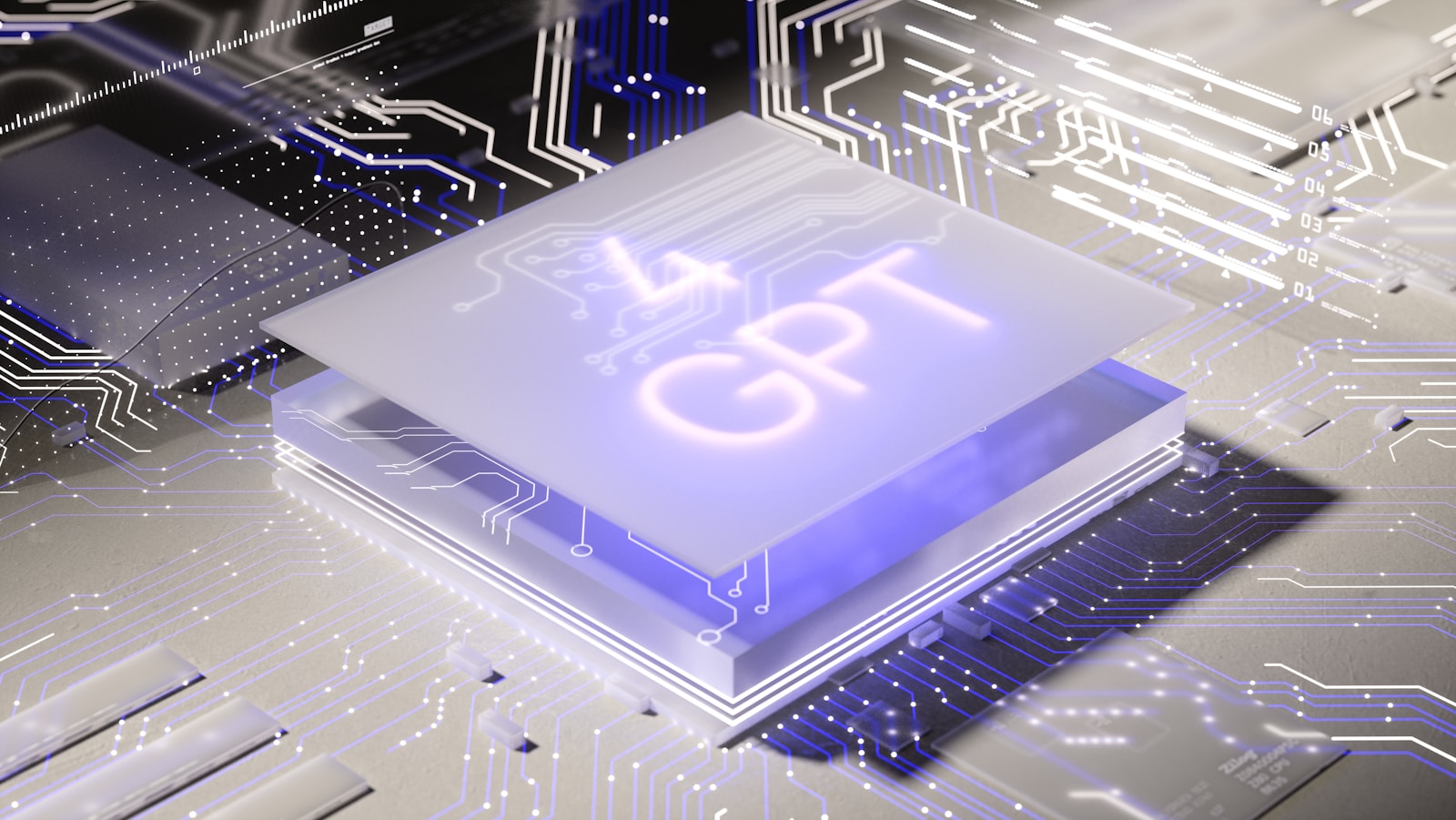
Insider Brief
- Terra Quantum introduced TQCompressor, an algorithm that shrinks large language models (LLMs) while maintaining comparable performance.
- The study demonstrates the compression of GPT-2 small, achieving a 35% reduction in the number of parameters.
- The significant time, computation and energy resources continue to rise for these large NLP models, particularly for training.
Terra Quantum, a leading quantum technology company, announced TQCompressor, an algorithm that shrinks large language models (LLMs) while maintaining comparable performance to address the growing demands of generative AI models.
The novel compression technique significantly reduces the size of datasets required for pre-training on targeted tasks compared to other widely used compression methods. The new case study demonstrates the compression of GPT-2 small, achieving a 35% reduction in the number of parameters. The compressed model also demonstrated superior speech generation capabilities relative to other prevalent compressed variants of this ChatGPT predecessor, despite employing up to 97% less data.
In the work, “TQCompressor: improving tensor decomposition methods in neural networks via permutations,” researchers compressed the benchmark model GPT-2 small from 117 million parameters to 81 million and evaluated its performance against other compressed models. When provided with various datasets, including a large collection of Wikipedia articles, the Terra Quantum model produced better results in predicting the next word in a sequence as well as generating coherent text based on contextual understanding.
“This compression algorithm can significantly lower the energy and compute costs of LLMs,” said Markus Pflitsch, CEO of Terra Quantum. “The advancement paves the way for neural network architecture optimizations that streamline GenAI to meet sustainability goals without compromising exceptional performance.”
GPT-2 small, the model presented in the paper, uses the same underpinning language architecture as GPT-2 and ChatGPT. GPT-2 has 1.5 billion parameters while the “small” version has 117 million parameters, representing the smallest of the GPT-2 versions released by OpenAI. Reducing the overall size of these LLMS opens up more use cases.
TQCompressor uses a tensor network technique to restructure connections between neurons of LLMs while preserving structural integrity. TQCompressedGPT-2, now publicly available on Hugging Face, is an advanced neural network model for natural language processing (NLP) tasks that achieves an improvement in efficiency and expressivity over GPT-2.
“When neural networks are compressed, they often lose expressivity – the ability to capture and represent complex patterns and relationships in data,” said Aleksei Naumov, AI Engineer at Terra Quantum and lead author of the paper. “Our optimization of the neural network enables a more effective compression process that mitigates loss in the model’s expressivity to deploy the AI model efficiently and effectively.”
The model also outperforms other GPT-2 compressions in perplexity scores, which measure how well language models accurately predict data, Naumov said. TQCompressedGPT-2 achieved better scores than popular compressed models like DistilGPT-2 across all benchmarking datasets.
The significant time, computation and energy resources required continue to rise for these large NLP models, particularly for training. Some researchers estimate that the carbon emissions from training a popular model exceed that of 6-passenger jet flights between San Francisco and New York. If every Google search integrated LLM-generated results, the annual electricity required could equal the consumption of Ireland.
Quantum-inspired techniques are a potential solution to this problem. TQCompressor reduced the training requirements of the model to prove the potential of tensor networks to streamline machine learning applications and develop more efficient LLMs, according to the company’s researchers.
Further research could explore the application of Terra Quantum’s compression techniques for larger use cases such as ChatGPT.
Generative AI offers the potential to transform industries across finance, healthcare, education and many more, and its impact can be further amplified with quantum computing. Quantum-inspired tensor network methods like TQCompressor open the door to transforming AI and NLP.